Modeling and Identification of ABE Fermentation Processes
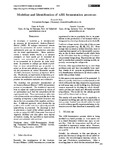
Use este enlace para citar
http://hdl.handle.net/2183/29590
A non ser que se indique outra cousa, a licenza do ítem descríbese como Atribución-NoComercial-CompartirIgual 4.0 Internacional
Coleccións
Metadatos
Mostrar o rexistro completo do ítemTítulo
Modeling and Identification of ABE Fermentation ProcessesData
2016Cita bibliográfica
Hose, D., Prada, C. de, González, G. Modeling and identification of ABE fermentation processes. En Actas de las XXXVII Jornadas de Automática. 7, 8 y 9 de septiembre de 2016, Madrid (pp. 278-285). DOI capítulo: https://doi.org/10.17979/spudc.9788497498081.0278 DOI libro: https://doi.org/10.17979/spudc.9788497498081
Resumo
[Resumen] Se investigan el modelado y la identificación de procesos de fermentación Acetona-Butanol-Etanol (ABE). El enfoque tradicional intenta ajustar los parametros del modelo mediante una optimización para que las simulacines coincidan con los datos experimentales. Estas optimizaciones a menudo tardan mucho y no siempre consiguen un buen ajuste por la necesidad de imponer una estructura de modelo fijo y por la no-convexidad de la función de coste resultante. Se presenta un enfoque que divide el problema en unos sub-problemas que se pueden resolver de forma más efectiva y que elige el modelo del crecimiento de celulas libremente usando ALAMO (Automatic Learning of Algebraic MOdels). Finalmente, se implementa el algoritmo y realiza una identificación con datos reales y se com- prueban los resultados mediante una validación. [Abstract] The modeling and parameter identification of an Acetone-Butanol-Ethanol (ABE) fermentation process is investigated. The traditional approach
tries to adjust the model parameters by means of an optimization such that the simulations fit the experimental data. These optimizations often take
a lot of time and do not achieve good fits due to the necessity of imposing a xed model structure and due to the non-convexity of the resulting cost function. A different approach, which divides the big problem into smaller and more efficiently solvable subproblems, is presented. Its advantage is that it determines a model of the cellular growth term on the go using ALAMO (Automatic Learning of Algebraic MOdels) and thus others more degrees of freedom. Lastly, the algorithm is implemented, tested and validated with real data.
Palabras chave
ABE Fermentation
Modeling
Parameter identification
Cellular Growth
Tikhonov Regularization
ALAMO
Modeling
Parameter identification
Cellular Growth
Tikhonov Regularization
ALAMO
Versión do editor
Dereitos
Atribución-NoComercial-CompartirIgual 4.0 Internacional
ISBN
978-84-617-4298-1 (UCM) 978-84-9749-808-1 (UDC electrónico)