Mapping the Poverty Proportion in Small Areas under Random Regression Coefficient Poisson Models
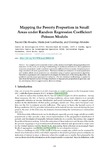
Use este enlace para citar
http://hdl.handle.net/2183/34236
A non ser que se indique outra cousa, a licenza do ítem descríbese como Attribution 4.0 International (CC BY 4.0)
Metadatos
Mostrar o rexistro completo do ítemTítulo
Mapping the Poverty Proportion in Small Areas under Random Regression Coefficient Poisson ModelsData
2023Resumo
[Abstract] In a complex socio-economic context, policy makers need highly disaggregated poverty indicators. In this work, we develop a methodology in small area estimation to derive predictors of poverty proportions under a random regression coefficient Poisson model, introducing bootstrap estimators of mean squared errors. Maximum likelihood estimators of model parameters and random effects mode predictors are calculated using a Laplace approximation algorithm. Simulation experiments are conducted to investigate the behaviour of the fitting algorithm, the predictors and the mean squared error estimator. The new statistical methodology is applied to data from the Spanish survey of living conditions to map poverty proportions by province and sex, developing a tool to support policy decision making
Palabras chave
Algoritmos de aproximación
Simulación
Pobreza
Simulación
Pobreza
Descrición
Cursos e Congresos, C-155
Versión do editor
Dereitos
Attribution 4.0 International (CC BY 4.0)