Distributed classification based on distances between probability distributions in feature space
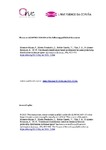
Use this link to cite
http://hdl.handle.net/2183/36030
Except where otherwise noted, this item's license is described as Atribución-NoComercial-SinDerivadas 4.0 Internacional
Collections
- GI-LIDIA - Artigos [62]
- GI-MODES - Artigos [139]
Metadata
Show full item recordTitle
Distributed classification based on distances between probability distributions in feature spaceAuthor(s)
Date
2019-09Citation
Montero-Manso, P., Morán-Fernández, L., Bolón-Canedo, V., Vilar, J. A., & Alonso-Betanzos, A. (2019). Distributed classification based on distances between probability distributions in feature space. Information Sciences, 496, 431–450. https://doi.org/10.1016/j.ins.2018.12.044
Abstract
[Abstract]: We consider a distributed framework where training and test samples drawn from the same distribution are available, with the training instances spread across disjoint nodes. In this setting, a novel learning algorithm based on combining with different weights the outputs of classifiers trained at each node is proposed. The weights depend on the distributional distance between each node and the test set in the feature space. Two different weighting approaches are introduced, which are referred to as per-Node Weighting (pNW) and per-Instance Weighting (pIW). While pNW assigns the same weight to all test instances at each node, pIW allows distinct weights for test instances differently represented at the node. By construction, our approach is particularly useful to deal with unbalanced nodes. Our methods require no communication between nodes, allowing for data privacy, independence of the kind of trained classifier at each node and maximum training speedup. In fact, our methods do not require retraining of the node’s classifiers if available. Although a range of different combination rules are considered to ensemble the single classifiers, theoretical support for the optimality of using the sum rule is provided. Our experiments illustrate all of these properties and show that pIW produces the highest classification accuracies compared with pNW and the standard unweighted approaches.
Keywords
Distributed classification
Distributional distances
Classifiers combination
Imbalanced data set
Classification accuracy
Distributional distances
Classifiers combination
Imbalanced data set
Classification accuracy
Description
© 2017. This manuscript version is made available under the CC-BY-NC-ND 4.0 license https://creativecommons.org/licenses/by-nc-nd/4.0/. This version of the article: Montero-Manso, P., Morán-Fernández, L., Bolón-Canedo, V., Vilar, J. A., & Alonso-Betanzos, A. (2019). ‘Distributed classification based on distances between probability distributions in feature space’ has been accepted for publication in: Information Sciences, 496, 431–450. The Version of Record is available online at https://doi.org/10.1016/j.ins.2018.12.044.
Editor version
Rights
Atribución-NoComercial-SinDerivadas 4.0 Internacional
ISSN
0020-0255