EEG Signal Processing with Separable Convolutional Neural Network for Automatic Scoring of Sleeping Stage
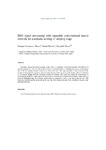
View/Open
Use this link to cite
http://hdl.handle.net/2183/25954
Except where otherwise noted, this item's license is described as Atribución-NoComercial-SinDerivadas 4.0 Interancional
Collections
- Investigación (FIC) [1705]
Metadata
Show full item recordTitle
EEG Signal Processing with Separable Convolutional Neural Network for Automatic Scoring of Sleeping StageDate
2020-06-01Citation
Fernandez-Blanco E, Rivero D, Pazos A. EEG signal processing with separable convolutional neural network for automatic scoring of sleeping stage. Neurocomputing. 2020; 410:220-228
Abstract
[Abstract]
Nowadays, among the Deep Learning works, there is a tendency to develop networks with millions of
trainable parameters. However, this tendency has two main drawbacks: overfitting and resource consumption due to the low-quality features extracted by those networks. This paper presents a study
focused on the scoring of sleeping EEG signals to measure if the increase of the pressure on the features
due to a reduction of the number though different techniques results in a benefit. The work also studies
the convenience of increasing the number of input signals in order to allow the network to extract better
features. Additionally, it might be highlighted that the presented model achieves comparable results to
the state-of-the-art with 1000 times less trainable and the presented model uses the whole dataset
instead of the simplified versions in the published literature.
Keywords
Convolutional neural networks
Deep learning
EEG
Signal processing
Sleep scoring
Deep learning
EEG
Signal processing
Sleep scoring
Editor version
Rights
Atribución-NoComercial-SinDerivadas 4.0 Interancional
ISSN
0925-2312