Finding a needle in a haystack: insights on feature selection for classification tasks
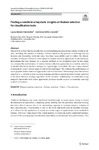
Use este enlace para citar
http://hdl.handle.net/2183/37634Coleccións
- Investigación (FIC) [1678]
Metadatos
Mostrar o rexistro completo do ítemTítulo
Finding a needle in a haystack: insights on feature selection for classification tasksData
2024-04Cita bibliográfica
Morán-Fernández, L., Bolón-Canedo, V. Finding a needle in a haystack: insights on feature selection for classification tasks. J Intell Inf Syst 62, 459-483 (2024). https://doi.org/10.1007/s10844-023-00823-y
Resumo
[Abstract]: The growth of Big Data has resulted in an overwhelming increase in the volume of data available, including the number of features. Feature selection, the process of selecting relevant features and discarding irrelevant ones, has been successfully used to reduce the dimensionality of datasets. However, with numerous feature selection approaches in the literature, determining the best strategy for a specific problem is not straightforward. In this study, we compare the performance of various feature selection approaches to a random selection to identify the most effective strategy for a given type of problem. We use a large number of datasets to cover a broad range of real-world challenges. We evaluate the performance of seven popular feature selection approaches and five classifiers. Our findings show that feature selection is a valuable tool in machine learning and that correlation-based feature selection is the most effective strategy regardless of the scenario. Additionally, we found that using improper thresholds with ranker approaches produces results as poor as randomly selecting a subset of features.
Palabras chave
Classification
Dimensionality reduction
Feature selection
Filters
Dimensionality reduction
Feature selection
Filters
Descrición
Financiado para publicación en acceso aberto: CRUE-CSIC/Springer Nature
Versión do editor
Dereitos
Atribución 3.0 España