A non-projective greedy dependency parser with bidirectional LSTMs
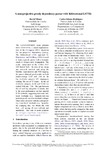
Ver/ abrir
Use este enlace para citar
http://hdl.handle.net/2183/34965
A non ser que se indique outra cousa, a licenza do ítem descríbese como Atribución 4.0 Internacional
Coleccións
- Investigación (FFIL) [853]
Metadatos
Mostrar o rexistro completo do ítemTítulo
A non-projective greedy dependency parser with bidirectional LSTMsData
2017-08Cita bibliográfica
David Vilares and Carlos Gómez-Rodríguez. 2017. A non-projective greedy dependency parser with bidirectional LSTMs. In Proceedings of the CoNLL 2017 Shared Task: Multilingual Parsing from Raw Text to Universal Dependencies, pages 152–162, Vancouver, Canada. Association for Computational Linguistics.
Resumo
[Abstract]: The LyS-FASTPARSE team present BIST-COVINGTON, a neural implementation of the Covington (2001) algorithm for non-projective dependency parsing. The bidirectional LSTM approach by Kiperwasser and Goldberg (2016) is used to train a greedy parser with a dynamic oracle to mitigate error propagation. The model participated in the CoNLL 2017 UD Shared Task. In spite of not using any ensemble methods and using the baseline segmentation and PoS tagging, the parser obtained good results on both macro-average LAS and UAS in the big treebanks category (55 languages), ranking 7th out of 33 teams. In the all treebanks category (LAS and UAS) we ranked 16th and 12th. The gap between the all and big categories is mainly due to the poor performance on four parallel PUD treebanks, suggesting that some ‘suffixed’ treebanks (e.g. Spanish-AnCora) perform poorly on cross-treebank settings, which does not occur with the corresponding ‘unsuffixed’ treebank (e.g. Spanish). By changing that, we obtain the 11th best LAS among all runs (official and unofficial). The code is made available at https://github.com/CoNLL-UD-2017/LyS-FASTPARSE.
Palabras chave
Multilingual Parsing
BIST-COVINGTON
Dependency parsing
Natural language processing
BIST-COVINGTON
Dependency parsing
Natural language processing
Versión do editor
Dereitos
Atribución 4.0 Internacional