A Deep Learning-Based Strategy to Predict Self-Interference in SFN DTT
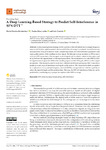
Use este enlace para citar
http://hdl.handle.net/2183/29351Coleccións
- Investigación (FIC) [1685]
Metadatos
Mostrar o rexistro completo do ítemTítulo
A Deep Learning-Based Strategy to Predict Self-Interference in SFN DTTData
2021Cita bibliográfica
Pereira-Ruisánchez, D.; Pérez-Adán, D.; Castedo, L. A Deep Learning-Based Strategy to Predict Self-Interference in SFN DTT. Eng. Proc. 2021, 7, 57. https://doi.org/10.3390/engproc2021007057
Resumo
[Abstract] A deep learning-based strategy for the analysis of the self-interference in single frequency networks (SFNs) for digital terrestrial television (DTT) broadcasting is considered. Several laboratory measurements were performed to create a dataset that relates the self-interference parameters and some quality metrics of the resulting received signal. The laboratory setup emulates an SFN scenario with two DTT transmitters. The strongest received signal and the relative values of attenuation and delay between the signals stand for the input parameters. The modulation error ratio (MER) of the strongest received signal, the MER of the resulting signal, and the SFN gain (SFNG) are the output parameters. This dataset is used to train four different multi-layer perceptron (MLP) models to predict accurate maps of interference and signal quality metrics. The considered models are suitable as complements for any multiple frequency network (MFN) coverage software with the capability to return the signal strength and the position data. This way, the SFN self-interference behavior can be predicted by considering only a proper description of the MFN coverage.
Palabras chave
SFN
Deep learning
Broadcasting
Self-interference
Deep learning
Broadcasting
Self-interference
Descrición
This article belongs to the Proceedings of The 4th XoveTIC Conference
Versión do editor
Dereitos
Atribución 3.0 España