Data Augmentation Approaches Using Cycle-Consistent Adversarial Networks for Improving COVID-19 Screening in Portable Chest X-Ray Images
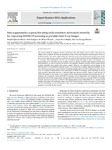
Use este enlace para citar
http://hdl.handle.net/2183/28802
A non ser que se indique outra cousa, a licenza do ítem descríbese como Atribución-NoComercial-SinDerivadas 4.0 Internacional
Coleccións
- Investigación (FIC) [1685]
Metadatos
Mostrar o rexistro completo do ítemTítulo
Data Augmentation Approaches Using Cycle-Consistent Adversarial Networks for Improving COVID-19 Screening in Portable Chest X-Ray ImagesData
2021Cita bibliográfica
MORÍS, Daniel Iglesias, et al. Data augmentation approaches using cycle-consistent adversarial networks for improving COVID-19 screening in portable chest X-ray images. Expert Systems with Applications, 2021, vol. 185, p. 115681. DOI: 10.1016/j.eswa.2021.115681
Resumo
[Abstract] The current COVID-19 pandemic, that has caused more than 100 million cases as well as more than two million deaths worldwide, demands the development of fast and accurate diagnostic methods despite the lack of available samples. This disease mainly affects the respiratory system of the patients and can lead to pneumonia and to severe cases of acute respiratory syndrome that result in the formation of several pathological structures in the lungs. These pathological structures can be explored taking advantage of chest X-ray imaging. As a recommendation for the health services, portable chest X-ray devices should be used instead of conventional fixed machinery, in order to prevent the spread of the pathogen. However, portable devices present several problems (specially those related with capture quality). Moreover, the subjectivity and the fatigue of the clinicians lead to a very difficult diagnostic process. To overcome that, computer-aided methodologies can be very useful even taking into account the lack of available samples that the COVID-19 affectation shows. In this work, we propose an improvement in the performance of COVID-19 screening, taking advantage of several cycle generative adversarial networks to generate useful and relevant synthetic images to solve the lack of COVID-19 samples, in the context of poor quality and low detail datasets obtained from portable devices. For validating this proposal for improved COVID-19 screening, several experiments were conducted. The results demonstrate that this data augmentation strategy improves the performance of a previous COVID-19 screening proposal, achieving an accuracy of 98.61% when distinguishing among NON-COVID-19 (i.e. normal control samples and samples with pathologies others than COVID-19) and genuine COVID-19 samples. It is remarkable that this methodology can be extrapolated to other pulmonary pathologies and even other medical imaging domains to overcome the data scarcity.
Palabras chave
X-ray portable device
COVID-19
Data augmentation
Screening
CycleGAN
Deep learning
COVID-19
Data augmentation
Screening
CycleGAN
Deep learning
Descrición
Financiado para publicación en acceso aberto: Universidade da Coruña/CISUG
Versión do editor
Dereitos
Atribución-NoComercial-SinDerivadas 4.0 Internacional