Estimating Lengths-Of-Stay of Hospitalized COVID-19 Patients Using a Non-parametric Model: A Case Study in Galicia (Spain)
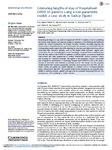
Use este enlace para citar
http://hdl.handle.net/2183/28123
A non ser que se indique outra cousa, a licenza do ítem descríbese como Atribución 4.0 Internacional
Coleccións
- GI-MODES - Artigos [126]
Metadatos
Mostrar o rexistro completo do ítemTítulo
Estimating Lengths-Of-Stay of Hospitalized COVID-19 Patients Using a Non-parametric Model: A Case Study in Galicia (Spain)Data
2021Cita bibliográfica
López-Cheda A, Jácome M-A, Cao R, De Salazar PM (2021). Estimating lengths-of-stay of hospitalised COVID-19 patients using a non-parametric model: a case study in Galicia (Spain). Epidemiology and Infection 149, e102, 1–8. https://doi.org/ 10.1017/S0950268821000959
Resumo
[Abstract] Estimating the lengths-of-stay (LoS) of hospitalised COVID-19 patients is key for predicting the hospital beds’ demand and planning mitigation strategies, as overwhelming the healthcare systems has critical consequences for disease mortality. However, accurately mapping the time-to-event of hospital outcomes, such as the LoS in the intensive care unit (ICU), requires understanding patient trajectories while adjusting for covariates and observation bias, such as incomplete data. Standard methods, such as the Kaplan-Meier estimator, require prior assumptions that are untenable given current knowledge. Using real-time surveillance data from the first weeks of the COVID-19 epidemic in Galicia (Spain), we aimed to model the time-to-event and event probabilities of patients’ hospitalised, without parametric priors and adjusting for individual covariates. We applied a non-parametric mixture cure model and compared its performance in estimating hospital ward (HW)/ICU LoS to the performances of commonly used methods to estimate survival. We showed that the proposed model outperformed standard approaches, providing more accurate ICU and HW LoS estimates. Finally, we applied our model estimates to simulate COVID-19 hospital demand using a Monte Carlo algorithm. We provided evidence that adjusting for sex, generally overlooked in prediction models, together with age is key for accurately forecasting HW and ICU occupancy, as well as discharge or death outcomes.
Palabras chave
COVID-19
Forecasting
ICU
Length-of-stay
Mixture cure model
Non-parametric
Forecasting
ICU
Length-of-stay
Mixture cure model
Non-parametric
Versión do editor
Dereitos
Atribución 4.0 Internacional