Automatic classification of respiratory patterns involving missing data imputation techniques
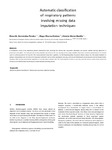
Use este enlace para citar
http://hdl.handle.net/2183/18101
A non ser que se indique outra cousa, a licenza do ítem descríbese como Atribución-NoComercial-SinDerivadas 3.0 España
Coleccións
- GI-LIDIA - Artigos [64]
Metadatos
Mostrar o rexistro completo do ítemTítulo
Automatic classification of respiratory patterns involving missing data imputation techniquesData
2015-10Cita bibliográfica
Elena M. Hernández-Pereira, Diego Álvarez-Estévez, Vicente Moret-Bonillo, Automatic classification of respiratory patterns involving missing data imputation techniques, Biosystems Engineering 138 (2015), pp. 65–76
Resumo
[Abstract] A comparative study of the respiratory pattern classification task, involving five missing data imputation techniques and several machine learning algorithms is
presented in this paper. The main goal was to find a classifier that achieves the best accuracy results using a scalable imputation method in comparison to the method
used in a previous work of the authors. The results obtained show that in general, the Self-Organising Map imputation method allows non-tree based classifiers to
achieve improvements over the rest of the imputation methods in terms of the classification accuracy, and that the Feedforward neural network and the Random Forest
classifiers offer the best performance regardless of the imputation method used. The improvements in terms of accuracy over the previous work of the authors are
limited but the Feed Forward neural network model achieves promising results.
Palabras chave
Respiratory pattern classification
Missing data imputation
Machine learning
Missing data imputation
Machine learning
Versión do editor
Dereitos
Atribución-NoComercial-SinDerivadas 3.0 España
ISSN
1537-5110
1537-5129
1537-5129