Robust deep learning-based approach for retinal layer segmentation in optical coherence tomography images
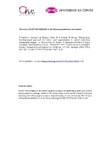
Use este enlace para citar
http://hdl.handle.net/2183/36809Coleccións
- Investigación (FIC) [1678]
Metadatos
Mostrar o rexistro completo do ítemTítulo
Robust deep learning-based approach for retinal layer segmentation in optical coherence tomography imagesAutor(es)
Data
2022Cita bibliográfica
A. Budiño, L. Ramos, J. de Moura, J. Novo, M. G. Penedo, M. Ortega, "Robust deep learning-based approach for retinal layer segmentation in optical coherence tomography images", In: Moreno-Díaz, R., Pichler, F., Quesada-Arencibia, A. (eds) Computer Aided Systems Theory – EUROCAST 2022. Lecture Notes in Computer Science, Revised Selected Papers, vol. 13789, pp. 427–434, Springer. ISBN: 978-3 031-25311-9, doi: 10.1007/978-3-031-25312-6_50
Resumo
[Abstract]: In recent years, the medical image analysis field has experienced remarkable growth. Advances in computational power have made it possible to create increasingly complex diagnostic support systems based on deep learning. In ophthalmology, optical coherence tomography (OCT) enables the capture of highly detailed images of the retinal morphology, being the reference technology for the analysis of relevant ocular structures. This paper proposes a new methodology for the automatic segmentation of the main retinal layers using OCT images. The system provides a useful tool that facilitates the clinical evaluation of key ocular structures, such as the choroid, vitreous humour or inner retinal layers, as potential computational biomarkers for the analysis of different neurodegenerative disorders, including multiple sclerosis and Alzheimer’s disease.
Palabras chave
Computational retinal biomarkers
Computer-aided diagnosis
Neurodegenerative disorders
Optical coherence tomography
Computer-aided diagnosis
Neurodegenerative disorders
Optical coherence tomography
Descrición
18th International Conference on Computer Aided Systems Theory, EUROCAST 2022,Las Palmas de Gran Canaria,20 February 2022 through 25 February 2022 This version of the article has been accepted for publication, after peer review and is subject to Springer Nature’s AM terms of use, but is not the Version of Record and does not reflect post-acceptance improvements, or any corrections. The Version of Record is available online at: https://doi.org/10.1007/978-3-031-25312-6_50
Versión do editor
Dereitos
©2022 The Author(s), under exclusive license to Springer Nature Switzerland AG