Prediction of compound-target interaction using several artificial intelligence algorithms and comparison with a consensus-based strategy
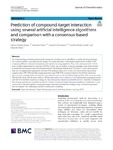
Ver/ abrir
Use este enlace para citar
http://hdl.handle.net/2183/36289Coleccións
- GI-RNASA - Artigos [177]
Metadatos
Mostrar o rexistro completo do ítemTítulo
Prediction of compound-target interaction using several artificial intelligence algorithms and comparison with a consensus-based strategyAutor(es)
Data
2024-03-07Cita bibliográfica
Jimenes-Vargas, K., Pazos, A., Munteanu, C.R. et al. Prediction of compound-target interaction using several artificial intelligence algorithms and comparison with a consensus-based strategy. J Cheminform 16, 27 (2024). https://doi.org/10.1186/s13321-024-00816-1
Resumo
[Absctract]: For understanding a chemical compound’s mechanism of action and its side effects, as well as for drug discovery, it is crucial to predict its possible protein targets. This study examines 15 developed target-centric models (TCM) employing different molecular descriptions and machine learning algorithms. They were contrasted with 17 third-party models implemented as web tools (WTCM). In both sets of models, consensus strategies were implemented as potential improvement over individual predictions. The findings indicate that TCM reach f1-score values greater than 0.8. Comparing both approaches, the best TCM achieves values of 0.75, 0.61, 0.25 and 0.38 for true positive/negative rates (TPR, TNR) and false negative/positive rates (FNR, FPR); outperforming the best WTCM. Moreover, the consensus strategy proves to have the most relevant results in the top
of target profiles. TCM consensus reach TPR and FNR values of 0.98 and 0; while on WTCM reach values of 0.75 and 0.24. The implemented computational tool with the TCM and their consensus strategy at: https://bioquimio.udla.edu.ec/tidentification01/. Scientific Contribution: We compare and discuss the performances of 17 public compound-target interaction prediction models and 15 new constructions. We also explore a compound-target interaction prioritization strategy using a consensus approach, and we analyzed the challenging involved in interactions modeling.
Palabras chave
Target identification
Target fishing
Ligan-based modeling
Machine learning
QSAR
Target fishing
Ligan-based modeling
Machine learning
QSAR
Descrición
This work was funded by Universidad de Las Américas and Universidade da Coruña.
Versión do editor
Dereitos
Atribución 3.0 España
ISSN
1758-2946