Unsupervised contrastive unpaired image generation approach for improving tuberculosis screening using chest X-ray images
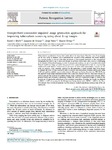
Use este enlace para citar
http://hdl.handle.net/2183/32221
A non ser que se indique outra cousa, a licenza do ítem descríbese como Atribución-NoComercial-SinDerivadas 3.0 España
Coleccións
- Investigación (FIC) [1634]
Metadatos
Mostrar o rexistro completo do ítemTítulo
Unsupervised contrastive unpaired image generation approach for improving tuberculosis screening using chest X-ray imagesData
2022-12Cita bibliográfica
D. I. Morís, J. de Moura, J. Novo, y M. Ortega, «Unsupervised contrastive unpaired image generation approach for improving tuberculosis screening using chest X-ray images», Pattern Recognition Letters, vol. 164, pp. 60-66, dic. 2022, doi: 10.1016/j.patrec.2022.10.026.
Resumo
[Abstract]: Tuberculosis is an infectious disease that mainly affects the lung tissues. Therefore, chest X-ray imaging can be very useful to diagnose and to understand the evolution of the pathology. This image modality has a poorer quality in contrast with other techniques as the magnetic resonance or the computerized tomography, but chest X-ray is easier and cheaper to perform. Furthermore, data scarcity is challenging in the domain of biomedical imaging. In order to mitigate this problem, the use of Generative Adversarial Network models for image generation has proved to be a powerful approach to train the deep learning models with small datasets, representing an alternative to classic data augmentation strategies. In this work, we propose a fully automatic approach for the generation of novel synthetic chest X-ray images to mitigate the effect of data scarcity in order to improve the tuberculosis screening performance using 3 different publicly available representative datasets: Montgomery County, Shenzhen and TBX11K. Firstly, this approach trains image translation models with a large-sized dataset (TBX11K). Then, these models are used to generate the novel set of synthetic images using small-sized and medium-sized datasets (Montgomery County and Shenzhen, respectively). Finally, the novel set of generated images is added to the training set to improve the performance of an automatic tuberculosis screening. As a result, we obtained an 88.41% 5.27% of accuracy for the Montgomery County dataset and a 90.33% 1.41% for the Shenzhen dataset. These results demonstrate that the proposed method outperforms previous state-of-the-art approaches.
Palabras chave
Tuberculosis
Chest X-ray
Deep learning
Biomedical imaging
Contrastive unpaired translation
Data scarcity
Chest X-ray
Deep learning
Biomedical imaging
Contrastive unpaired translation
Data scarcity
Dereitos
Atribución-NoComercial-SinDerivadas 3.0 España
ISSN
0167-8655