UAV Swarm Path Planning With Reinforcement Learning for Field Prospecting
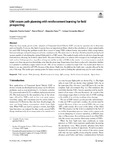
Ver/ abrir
Use este enlace para citar
http://hdl.handle.net/2183/29921
A non ser que se indique outra cousa, a licenza do ítem descríbese como Atribución 4.0 Internacional
Coleccións
- GI-RNASA - Artigos [195]
- INIBIC-RNASA-IMEDIR - Artigos [46]
Metadatos
Mostrar o rexistro completo do ítemTítulo
UAV Swarm Path Planning With Reinforcement Learning for Field ProspectingData
2022-03-03Cita bibliográfica
Puente-Castro, A., Rivero, D., Pazos, A. et al. UAV swarm path planning with reinforcement learning for field prospecting. Appl Intell (2022). https://doi.org/10.1007/s10489-022-03254-4
Resumo
[Abstract] There has been steady growth in the adoption of Unmanned Aerial Vehicle (UAV) swarms by operators due to their time and cost benefits. However, this kind of system faces an important problem, which is the calculation of many optimal paths for each UAV. Solving this problem would allow control of many UAVs without human intervention while saving battery between recharges and performing several tasks simultaneously. The main aim is to develop a Reinforcement Learning based system capable of calculating the optimal flight path for a UAV swarm. This method stands out for its ability to learn through trial and error, allowing the model to adjust itself. The aim of these paths is to achieve full coverage of an overflight area for tasks such as field prospection, regardless of map size and the number of UAVs in the swarm. It is not necessary to establish targets or to have any previous knowledge other than the given map. Experiments have been conducted to determine whether it is optimal to establish a single control for all UAVs in the swarm or a control for each UAV. The results show that it is better to use one control for all UAVs because of the shorter flight time. In addition, the flight time is greatly affected by the size of the map. The results give starting points for future research, such as finding the optimal map size for each situation.
Palabras chave
UAV swarm
Path planning
Reinforcement learning
Q-Learning
Artificial neural network
Agriculture
Path planning
Reinforcement learning
Q-Learning
Artificial neural network
Agriculture
Descrición
Financiado para publicación en acceso aberto: Universidade da Coruña/CISUG
Versión do editor
Dereitos
Atribución 4.0 Internacional
ISSN
0924-669X