Improving Medical Data Annotation Including Humans in the Machine Learning Loop
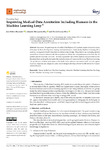
Use este enlace para citar
http://hdl.handle.net/2183/29300
A non ser que se indique outra cousa, a licenza do ítem descríbese como Atribución 4.0 Internacional
Coleccións
Metadatos
Mostrar o rexistro completo do ítemTítulo
Improving Medical Data Annotation Including Humans in the Machine Learning LoopData
2021Cita bibliográfica
Bobes-Bascarán, J.; Mosqueira-Rey, E.; Alonso-Ríos, D. Improving Medical Data Annotation Including Humans in the Machine Learning Loop. Eng. Proc. 2021, 7, 39. https://doi.org/10.3390/engproc2021007039
Resumo
[Abstract] At present, the great majority of Artificial Intelligence (AI) systems require the participation of humans in their development, tuning, and maintenance. Particularly, Machine Learning (ML) systems could greatly benefit from their expertise or knowledge. Thus, there is an increasing interest around how humans interact with those systems to obtain the best performance for both the AI system and the humans involved. Several approaches have been studied and proposed in the literature that can be gathered under the umbrella term of Human-in-the-Loop Machine Learning. The application of those techniques to the health informatics environment could provide a great value on prognosis and diagnosis tasks contributing to develop a better health service for Cancer related diseases.
Palabras chave
Human-in-the-Loop
Machine learning
Interactive Machine Learning
Machine Teaching
Iterative Machine Teaching
Active learning
Machine learning
Interactive Machine Learning
Machine Teaching
Iterative Machine Teaching
Active learning
Descrición
Presented at the 4th XoveTIC Conference, A Coruña, Spain, 7–8 October 2021
Versión do editor
Dereitos
Atribución 4.0 Internacional
Ítems relacionados
Mostrando ítems relacionados por Título, autor ou materia.
-
Integrating Iterative Machine Teaching and Active Learning into the Machine Learning Loop
Mosqueira-Rey, E.; Alonso Ríos, David; Baamonde-Lozano, Andrés (Elsevier, 2021)[Abstract] Scholars and practitioners are defining new types of interactions between humans and machine learning algorithms that we can group under the umbrella term of Human-in-the-Loop Machine Learning (HITL-ML). This ... -
A scalable decision-tree-based method to explain interactions in dyadic data
Eiras-Franco, Carlos; Guijarro-Berdiñas, Bertha; Alonso-Betanzos, Amparo; Bahamonde, Antonio (Elsevier, 2019-12)[Abstract]: Gaining relevant insight from a dyadic dataset, which describes interactions between two entities, is an open problem that has sparked the interest of researchers and industry data scientists alike. However, ... -
Human-in-the-loop machine learning: a state of the art
Mosqueira-Rey, E.; Hernández-Pereira, Elena; Alonso Ríos, David; Bobes-Bascarán, José; Fernández-Leal, Ángel (Springer Nature, 2023-04)[Abstract]: Researchers are defining new types of interactions between humans and machine learning algorithms generically called human-in-the-loop machine learning. Depending on who is in control of the learning process, ...