OpenCNN: A Winograd Minimal Filtering Algorithm Implementation in CUDA
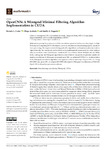
Use este enlace para citar
http://hdl.handle.net/2183/28443
A non ser que se indique outra cousa, a licenza do ítem descríbese como Atribución 4.0 Internacional
Coleccións
- Investigación (FIC) [1656]
Metadatos
Mostrar o rexistro completo do ítemTítulo
OpenCNN: A Winograd Minimal Filtering Algorithm Implementation in CUDAData
2021Cita bibliográfica
Castro, R.L.; Andrade, D.; Fraguela, B.B. OpenCNN: A Winograd Minimal Filtering Algorithm Implementation in CUDA. Mathematics 2021, 9, 2033. https://doi.org/10.3390/math9172033
Resumo
[Abstract] Improving the performance of the convolution operation has become a key target for High Performance Computing (HPC) developers due to its prevalence in deep learning applied mainly to video processing. The improvement is being pushed by algorithmic and implementation innovations. Algorithmically, the convolution can be solved as it is mathematically enunciated, but other methods allow to transform it into a Fast Fourier Transform (FFT) or a GEneral Matrix Multiplication (GEMM). In this latter group, the Winograd algorithm is a state-of-the-art variant that is specially suitable for smaller convolutions. In this paper, we present openCNN, an optimized CUDA C++ implementation of the Winograd convolution algorithm. Our approach achieves speedups of up to 1.76× on Turing RTX 2080Ti and up to 1.85× on Ampere RTX 3090 with respect to Winograd convolution in cuDNN 8.2.0. OpenCNN is released as open-source software.
Palabras chave
Deep learning
Convolution
Winograd
CUDA
Convolution
Winograd
CUDA
Versión do editor
Dereitos
Atribución 4.0 Internacional