Visual complexity modelling based on image features fusion of multiple kernels
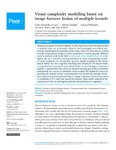
Use this link to cite
http://hdl.handle.net/2183/23991Collections
- Investigación (FIC) [1685]
Metadata
Show full item recordTitle
Visual complexity modelling based on image features fusion of multiple kernelsAuthor(s)
Date
2019-07-18Citation
Fernandez-Lozano C, Carballal A, Machado P, Santos A, Romero J. 2019. Visual complexity modelling based on image features fusion of multiple kernels. PeerJ 7:e7075 https://doi.org/10.7717/peerj.7075
Abstract
[Abstract] Humans’ perception of visual complexity is often regarded as one of the key principles of aesthetic order, and is intimately related to the physiological, neurological and, possibly, psychological characteristics of the human mind. For these reasons, creating accurate computational models of visual complexity is a demanding task. Building upon on previous work in the field (Forsythe et al., 2011; Machado et al., 2015) we explore the use of Machine Learning techniques to create computational models of visual complexity. For that purpose, we use a dataset composed of 800 visual stimuli divided into five categories, describing each stimulus by 329 features based on edge detection, compression error and Zipf’s law. In an initial stage, a comparative analysis of representative state-of-the-art Machine Learning approaches is performed. Subsequently, we conduct an exhaustive outlier analysis. We analyze the impact of removing the extreme outliers, concluding that Feature Selection Multiple Kernel Learning obtains the best results, yielding an average correlation to humans’ perception of complexity of 0.71 with only twenty-two features. These results outperform the current state-of-the-art, showing the potential of this technique for regression.
Keywords
Psychiatry and Psychology
Human–Computer Interaction
Computational Science
Data Mining and Machine Learning
Correlation
Machine learning
Zipf’s law
Compression error
Visual stimuli
Visual complexity
Human–Computer Interaction
Computational Science
Data Mining and Machine Learning
Correlation
Machine learning
Zipf’s law
Compression error
Visual stimuli
Visual complexity
Editor version
Rights
Atribución 3.0 España
ISSN
2167-8359