Anomaly Prediction in Cybersecurity: A Machine Learning Model from the Perspective of Data Engineering and Fingerprinting
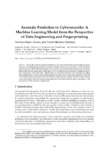
Use este enlace para citar
http://hdl.handle.net/2183/41130Metadatos
Mostrar el registro completo del ítemTítulo
Anomaly Prediction in Cybersecurity: A Machine Learning Model from the Perspective of Data Engineering and FingerprintingFecha
2024Resumen
Abstract: This project utilizes artificial intelligence (AI) and machine learning through the development
of a mathematical-predictive model to reliably detect cyber anomalies. Using the BETH
dataset and the CRISP-DM methodology, this research has addressed the problem of combining
kernel and network traffic data, achieving a 37.27% increase in the detection of malicious activities
compared to the initial data. Additionally, an innovative dataset was formulated, in which
the complete trace of a new botnet attack pattern was discovered, previously unknown to BETH,
involving the entire monitored network in illicit cryptocurrency mining. Finally, several models
were successfully built and trained using Random Forest and Decision Trees algorithms, with
accuracies of 100% and 99%, respectively.
Palabras clave
Artificial intelligence (AI)
Cybercriminals
Safeguards
Botnet attack pattern
Machine learning
Cybercriminals
Safeguards
Botnet attack pattern
Machine learning
Versión del editor
Derechos
Atribución 4.0