3D Features Fusion for Automated Segmentation of Fluid Regions in CSCR Patients: An OCT-based Photodynamic Therapy Response Analysis
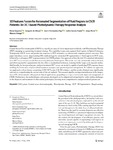
Use this link to cite
http://hdl.handle.net/2183/40479Collections
- Investigación (FIC) [1681]
Metadata
Show full item recordTitle
3D Features Fusion for Automated Segmentation of Fluid Regions in CSCR Patients: An OCT-based Photodynamic Therapy Response AnalysisAuthor(s)
Date
2024Citation
Goyanes, E., de Moura, J., Fernández-Vigo, J.I. et al. 3D Features Fusion for Automated Segmentation of Fluid Regions in CSCR Patients: An OCT-based Photodynamic Therapy Response Analysis. J Digit Imaging. Inform. med. (2024). https://doi.org/10.1007/s10278-024-01190-y
Abstract
[Abstract]: Central Serous Chorioretinopathy (CSCR) is a significant cause of vision impairment worldwide, with Photodynamic Therapy (PDT) emerging as a promising treatment strategy. The capability to precisely segment fluid regions in Optical Coherence Tomography (OCT) scans and predict the response to PDT treatment can substantially augment patient outcomes. This paper introduces a novel deep learning (DL) methodology for automated 3D segmentation of fluid regions in OCT scans, followed by a subsequent PDT response analysis for CSCR patients. Our approach utilizes the rich 3D contextual information from OCT scans to train a model that accurately delineates fluid regions. This model not only substantially reduces the time and effort required for segmentation but also offers a standardized technique, fostering further large-scale research studies. Additionally, by incorporating pre- and post-treatment OCT scans, our model is capable of predicting PDT response, hence enabling the formulation of personalized treatment strategies and optimized patient management. To validate our approach, we employed a robust dataset comprising 2,769 OCT scans (124 3D volumes), and the results obtained were significantly satisfactory, outperforming the current state-of-the-art methods. This research signifies an important milestone in the integration of DL advancements with practical clinical applications, propelling us a step closer towards improved management of CSCR. Furthermore, the methodologies and systems developed can be adapted and extrapolated to tackle similar challenges in the diagnosis and treatment of other retinal pathologies, favoring more comprehensive and personalized patient care.
Keywords
CAD system
Central serous chorioretinopathy
Photodynamic Therapy
OCT
3D Segmentation
Deep Learning
Central serous chorioretinopathy
Photodynamic Therapy
OCT
3D Segmentation
Deep Learning
Description
Financiado para publicación en acceso aberto: Universidade da Coruña/CISUG
Editor version
Rights
Atribución 3.0 España
ISSN
2948-2933