DSVD-autoencoder: A scalable distributed privacy-preserving method for one-class classification
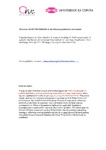
Use this link to cite
http://hdl.handle.net/2183/40142Collections
- Investigación (FIC) [1634]
Metadata
Show full item recordTitle
DSVD-autoencoder: A scalable distributed privacy-preserving method for one-class classificationDate
2021-01Citation
Fontenla-Romero O, Pérez-Sánchez B, Guijarro-Berdiñas B. DSVD-autoencoder: A scalable distributed privacy-preserving method for one-class classification. Int J Intell Syst. 2021; 36: 177-199. https://doi.org/10.1002/int.22296
Abstract
[Abstract]: One-class classification has gained interest as a solution to certain kinds of problems typical in a wide variety of real environments like anomaly or novelty detection. Autoencoder is the type of neural network that has been widely applied in these one-class problems. In the Big Data era, new challenges have arisen, mainly related with the data volume. Another main concern derives from Privacy issues when data is distributed and cannot be shared among locations. These two conditions make many of the classic and brilliant methods not applicable. In this paper, we present distributed singular value decomposition (DSVD-autoencoder), a method for autoencoders that allows learning in distributed scenarios without sharing raw data. Additionally, to guarantee privacy, it is noniterative and hyperparameter-free, two interesting characteristics when dealing with Big Data. In comparison with the state of the art, results demonstrate that DSVD-autoencoder provides a highly competitive solution to deal with very large data sets by reducing training from several hours to seconds while maintaining good accuracy.
Keywords
Autoencoder
Big data
Distributed learning
Neural network
One-class classification
Privacy-preserving
Singular value decomposition
Big data
Distributed learning
Neural network
One-class classification
Privacy-preserving
Singular value decomposition
Description
This is the peer reviewed version of the following article: DSVD-autoencoder: A scalable distributed privacy-preserving method for one-class classification, which has been published in final form at https://doi.org/10.1002/int.22296. This article may be used for non-commercial purposes in accordance with Wiley Terms and Conditions for Use of Self-Archived Versions. This article may not be enhanced, enriched or otherwise transformed into a derivative work, without express permission from Wiley or by statutory rights under applicable legislation. Copyright notices must not be removed, obscured or modified. The article must be linked to Wiley’s version of record on Wiley Online Library and any embedding, framing or otherwise making available the article or pages thereof by third parties from platforms, services and websites other than Wiley Online Library must be prohibited.
Editor version
Rights
© 2020 Wiley Periodicals LLC
ISSN
0884-8173