Deep Learning Methods to Mitigate Human-Factor-Related Accidents in Maritime Transport
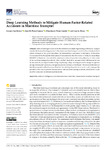
Use este enlace para citar
http://hdl.handle.net/2183/40018
Excepto si se señala otra cosa, la licencia del ítem se describe como Creative Commons Attribution (CC BY) license 4.0
Colecciones
- Investigación (EPEF) [590]
Metadatos
Mostrar el registro completo del ítemTítulo
Deep Learning Methods to Mitigate Human-Factor-Related Accidents in Maritime TransportFecha
2024Cita bibliográfica
Cao-Feijóo, G.; PérezCanosa, J.M.; Pérez-Castelo, F.J.; Orosa, J.A. Deep Learning Methods to Mitigate Human-Factor-Related Accidents in Maritime Transport. J. Mar. Sci. Eng. 2024, 12, 1819. https:// doi.org/10.3390/jmse12101819
Resumen
[Abstract] Artificial intelligence aims to be the solution to multiple engineering problems by trying to emulate the human learning process. In this sense, maritime transport standards have clearly evolved, which are based on two principal pillars: the International Convention for the Safety of Life at Sea Convention (SOLAS) and the International Convention for the Prevention of Pollution from Ships (MARPOL). Based on a formal safety assessment research process, these pillars try to solve most of the maritime transport accidents, which, in their final steps, are associated with human factors. In this research, an original methodology employing a deep learning process for image recognition during mooring line operation, a dangerous process on ships, is developed. The main results indicate that the proposed method is an excellent tool for advising ship officers on watch and, consequently, provides a new way to prevent human factors onboard from causing accidents, which in the future must be considered in international standards.
Palabras clave
Artificial intelligence
Convolutional neural networks
Human factor
Maritime transport
Convolutional neural networks
Human factor
Maritime transport
Versión del editor
Derechos
Creative Commons Attribution (CC BY) license 4.0