Clustering of Gene Expression Profiles Applied to Marine Research
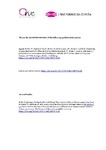
View/ Open
Use this link to cite
http://hdl.handle.net/2183/39887Collections
- Investigación (FIC) [1685]
Metadata
Show full item recordTitle
Clustering of Gene Expression Profiles Applied to Marine ResearchAuthor(s)
Date
2013Citation
Aguiar-Pulido, V., Suárez-Ulloa, V., Rivero, D., Eirín-López, J.M., Dorado, J. (2013). Clustering of Gene Expression Profiles Applied to Marine Research. In: Rojas, I., Joya, G., Gabestany, J. (eds) Advances in Computational Intelligence. IWANN 2013. Lecture Notes in Computer Science, vol 7902. Springer, Berlin, Heidelberg. https://doi.org/10.1007/978-3-642-38679-4_45
Abstract
[Abstract]: This work presents the results of applying two clustering techniques to gene expression data from the mussel Mytilus galloprovincialis. The objective of the study presented in this paper was to cluster the different genes involved in the experiment, in order to find those most closely related based on their expression patterns. A self-organising map (SOM) and the k-means algorithm were used, partitioning the input data into nine clusters. The resulting clusters were then analysed using Gene Ontology (GO) data, obtaining results that suggest that SOM clusters could be more homogeneous than those obtained by the k-means technique.
Keywords
Clustering
Microarray
Neural networks
Data mining
Bioinformatics
Gene ontology
Microarray
Neural networks
Data mining
Bioinformatics
Gene ontology
Description
This version of the conference paper has been accepted for publication, after peer review and is subject to Springer Nature’s AM terms of use (https://www.springernature.com/gp/open-science/policies/accepted-manuscript-terms), but is not the Version of Record and does not reflect post-acceptance improvements, or any corrections. The Version of Record is available online at: https://doi.org/10.1007/978-3-642-38679-4_45. Conference paper presented at: 12th International Work-Conference on Artificial Neural Networks, IWANN 2013, Puerto de la Cruz, Tenerife, Spain, June 12-14, 2013.
Editor version
Rights
© 2013 Springer-Verlag Berlin Heidelberg. Subject to Springer Nature’s AM terms of use (https://www.springernature.com/gp/open-science/policies/accepted-manuscript-terms).
ISSN
0302-9743
ISBN
978-3-642-38678-7