Efficient clinical decision-making process via AI-based multimodal data fusion: A COVID-19 case study
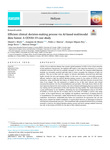
Use this link to cite
http://hdl.handle.net/2183/39569
Except where otherwise noted, this item's license is described as Attribution 4.0 International (CC BY)
Collections
- Investigación (FIC) [1678]
Metadata
Show full item recordTitle
Efficient clinical decision-making process via AI-based multimodal data fusion: A COVID-19 case studyAuthor(s)
Date
2024-10Citation
D. I. Morís, J. de Moura, P. J. Marcos, E. Míguez Rey, J. Novo, and M. Ortega, "Efficient clinical decision-making process via AI-based multimodal data fusion: A COVID-19 case study", Heliyon, vol. 10, n. 20, e38642, 2024, https://doi.org/10.1016/j.heliyon.2024.e38642
Abstract
[Abstract]: COVID-19 is an infectious disease that caused a global pandemic in 2020. In the critical moments of this healthcare emergencies, the medical staff needs to take important decisions in a context of limited resources that must be carefully managed. To this end, the computer-aided diagnosis methods are extremely powerful and help them to better recognize the evidences of high-risk patients. This can be done with the support of relevant information extracted from electronic
health records, lab tests and imaging studies. In this work, we present a novel fully-automatic efficient method to help the clinical decision-making process in the context of COVID-19 risk estimation, using multimodal data fusion of clinical features and deep features extracted from chest X-ray images. The risk estimation is studied in two of the most relevant and critical encountered scenarios: the risk of hospitalization and mortality. This study shows which are the most important features for each scenario, the ratio of clinical and imaging features present in the top ranking and the performance of the used machine learning models. The results demonstrate a great performance by the classifiers, estimating the risk of hospitalization with an AUC-ROC of 0.8452 ± 0.0133 and the risk of death with an AUC-ROC of 0.8285 ± 0.0210, only using
a subset of the original features, and highlight the significant contribution of imaging features to hospitalization risk assessment, while clinical features become more crucial for mortality risk evaluation. Furthermore, multimodal data fusion can outperform the approaches that use one data source. Despite the model’s complexity, it requires fewer features, an advantage in scenarios with limited computational resources. This streamlined, fully-automated method shows promising potential to improve the clinical decision-making process and better manage medical resources, not only in the context of COVID-19, but also in other clinical scenarios.
Keywords
Information fusion
Risk estimation
Clinical data
Deep features
COVID-19
Chest X-ray
Risk estimation
Clinical data
Deep features
COVID-19
Chest X-ray
Editor version
Rights
Attribution 4.0 International (CC BY)