Multistage strategy for ground point filtering on large-scale datasets
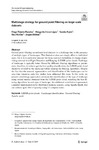
Use this link to cite
http://hdl.handle.net/2183/38935Collections
- Investigación (FIC) [1615]
Metadata
Show full item recordTitle
Multistage strategy for ground point filtering on large-scale datasetsDate
2024-08Citation
Teijeiro Paredes, D., Amor López, M., Buján, S. et al. Multistage strategy for ground point filtering on large-scale datasets. J Supercomput (2024). https://doi.org/10.1007/s11227-024-06406-0
Abstract
[Abstract]: Ground point filtering on national-level datasets is a challenge due to the presence of multiple types of landscapes. This limitation does not simply affect to individual users, but it is in particular relevant for those national institutions in charge of providing national-level Light Detection and Ranging (LiDAR) point clouds. Each type of landscape is typically better filtered by different filtering algorithms or parameters; therefore, in order to get the best quality classification, the LiDAR point cloud should be divided by the landscape before running the filtering algorithms. Despite the fact that the manual segmentation and identification of the landscapes can be very time intensive, only few studies have addressed this issue. In this work, we present a multistage approach to automate the identification of the type of landscape using several metrics extracted from the LiDAR point cloud, matching the best filtering algorithms in each type of landscape. An additional contribution is presented, a parallel implementation for distributed memory systems, using Apache Spark, that can achieve up to 34 x of speedup using 12 compute nodes.
Keywords
LiDAR point clouds
Landscape identification
Ground filtering
Apache spark
Landscape identification
Ground filtering
Apache spark
Description
The point clouds and LiDAR datasets used in this work belong to the LiDAR-PNOA data repository, region of Navarra, and were provided by Gobierno de Navarra. Dataset license: LiDAR-PNOA-cob2 2017 CC-BY 4.0 scne.es.
Editor version
Rights
Atribución 4.0 Internacional © 2024, The Authors
ISSN
0920-8542
1573-0484
1573-0484