PlayNet: real-time handball play classification with Kalman embeddings and neural networks
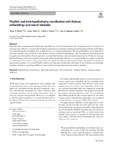
Use este enlace para citar
http://hdl.handle.net/2183/37536Colecciones
- Investigación (FIC) [1615]
Metadatos
Mostrar el registro completo del ítemTítulo
PlayNet: real-time handball play classification with Kalman embeddings and neural networksFecha
2024Cita bibliográfica
Mures, O.A., Taibo, J., Padrón, E.J. et al. (2023) PlayNet: real-time handball play classification with Kalman embeddings and neural networks. Vis Comput 40 (4), 2695–2711
Resumen
[Abstract] Real-time play recognition and classification algorithms are crucial for automating video production and live broadcasts of sporting events. However, current methods relying on human pose estimation and deep neural networks introduce high latency on commodity hardware, limiting their usability in low-cost real-time applications. We present PlayNet, a novel approach toreal-time handball play classification. Our method is based on Kalman embeddings, a new low-dimensional representation for game states that enables efficient operation on commodity hardware and customized camera layouts. Firstly, we leverage
Kalman filtering to detect and track the main agents in the playing field, allowing us to represent them in a single normalized coordinate space. Secondly,weutilize a neural network trained in nonlinear dimensionality reduction through fuzzy topological data structure analysis. As a result, PlayNet achieves real-time play classification with under 55 ms of latency on commodity hardware, making it a promising addition to automated live broadcasting and game analysis pipelines.
Palabras clave
Handball play classification
Real-time multimedia
Neural networks
Kalman filtering
Dimensionality reduction
Real-time multimedia
Neural networks
Kalman filtering
Dimensionality reduction
Descripción
Open Access funding provided thanks to the CRUE-CSIC agreement with Springer Nature
Versión del editor
Derechos
Atribución 4.0 Internacional
ISSN
0178-2789