Assessment of Pre-Trained Models Across Languages and Grammars
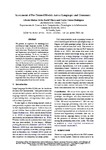
Use este enlace para citar
http://hdl.handle.net/2183/36572Coleccións
- OpenAIRE [313]
- GI-LYS - Congresos, conferencias, etc. [61]
Metadatos
Mostrar o rexistro completo do ítemTítulo
Assessment of Pre-Trained Models Across Languages and GrammarsData
2023-11Cita bibliográfica
Alberto Muñoz-Ortiz, David Vilares, and Carlos Gómez-Rodríguez. 2023. Assessment of Pre-Trained Models Across Languages and Grammars. In Proceedings of the 13th International Joint Conference on Natural Language Processing and the 3rd Conference of the Asia-Pacific Chapter of the Association for Computational Linguistics (Volume 1: Long Papers), pages 359–373, Nusa Dua, Bali. Association for Computational Linguistics.
Resumo
[Absctract]: We present an approach for assessing how
multilingual large language models (LLMs)
learn syntax in terms of multi-formalism syntactic structures. We aim to recover constituent
and dependency structures by casting parsing
as sequence labeling. To do so, we select a
few LLMs and study them on 13 diverse UD
treebanks for dependency parsing and 10 treebanks for constituent parsing. Our results show
that: (i) the framework is consistent across encodings, (ii) pre-trained word vectors do not
favor constituency representations of syntax
over dependencies, (iii) sub-word tokenization
is needed to represent syntax, in contrast to
character-based models, and (iv) occurrence
of a language in the pretraining data is more
important than the amount of task data when
recovering syntax from the word vectors.
Palabras chave
Syntax learning
Sequence labeling
Subword tokenization
Pre-trained word vectors
Language occurrence in pretraining data
Sequence labeling
Subword tokenization
Pre-trained word vectors
Language occurrence in pretraining data
Descrición
Bali, Indonesia. November, 1-4 2023.
Versión do editor
Dereitos
Atribución 3.0 España