Comprehensive Analysis of the Screening of COVID-19 Approaches in Chest X-ray Images from Portable Devices
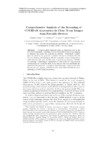
Use este enlace para citar
http://hdl.handle.net/2183/36556Colecciones
- Investigación (FIC) [1685]
Metadatos
Mostrar el registro completo del ítemTítulo
Comprehensive Analysis of the Screening of COVID-19 Approaches in Chest X-ray Images from Portable DevicesFecha
2021Cita bibliográfica
D. I. Morís, J. de Moura, J. Novo and M. Ortega, "Comprehensive Analysis of the Screening of COVID-19 Approaches in Chest X-ray Images from Portable Devices", ESANN 2021 proceedings, 29th European Symposium on Artificial Neural Networks, Computational Intelligence and Machine Learning. Online event, 6-8 October 2021, ISBN 978287587082-7, pp. 165-170. doi: https://doi.org/10.14428/esann/2021.ES2021-31
Resumen
[Abstract]: Computer-aided diagnosis plays an important role in the COVID-19 pandemic. Currently, it is recommended to use X-ray imaging to diagnose and assess the evolution in patients. Particularly, radiologists are asked to use portable acquisition devices to minimize the risk of cross-infection, facilitating an effective separation of suspected patients with other low-risk cases. In this work, we present an automatic COVID-19 screening, considering 6 representative state-of-the-art deep network architectures on a portable chest X-ray dataset that was specifically designed for this proposal. Exhaustive experimentation demonstrates that the models can separate COVID-19 cases from NON-COVID-19 cases, achieving a 97.68% of global accuracy.
Palabras clave
Computer aided diagnosis
Network architecture
Network architecture
Descripción
ESANN 2021 Proceedings - 29th European Symposium on Artificial Neural Networks, Computational Intelligence and Machine LearningOpen AccessPages 165 - 1702021 29th European Symposium on Artificial Neural Networks, Computational Intelligence and Machine Learning, ESANN 2021Virtual, Online 6 October 2021 through 8 October 2021 Code 178821
Versión del editor
Derechos
© 2021 ESANN Intelligence and Machine Learning. All rights reserved.