Context encoder self-supervised approaches for eye fundus analysis
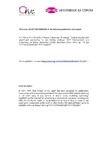
Use este enlace para citar
http://hdl.handle.net/2183/36500Colecciones
- Investigación (FIC) [1679]
Metadatos
Mostrar el registro completo del ítemTítulo
Context encoder self-supervised approaches for eye fundus analysisAutor(es)
Fecha
2021Cita bibliográfica
D. I. Morís, Á. S. Hervella, J. Rouco, J. Novo and M. Ortega, "Context encoder self-supervised approaches for eye fundus analysis," 2021 International Joint Conference on Neural Networks (IJCNN), Shenzhen, China, 2021, pp. 1-8, doi: 10.1109/IJCNN52387.2021.9533567
Resumen
[Abstract]: The broad availability of medical images in current clinical practice provides a source of large image datasets. In order to use these datasets for training deep neural networks in detection and segmentation tools, it is necessary to provide pixel-wise annotations associated to each image. However, the image annotation is a tedious, time consuming and error prone process that requires the participation of experienced specialists. In this work, we propose different complementary context encoder self-supervised approaches to learn relevant characteristics for the restricted medical imaging domain of retinographies. In particular, we propose a patch-wise approach, inspired in the previous proposal of broad domain context encoders, and complementary fully convolutional approaches. These approaches take advantage of the restricted application domain to learn the relevant features of the eye fundus, situation that can be extrapolated to many medical imaging issues. Different representative experiments were conducted in order to evaluate the performance of the trained models, demonstrating the suitability of the proposed approaches in the understanding of the eye fundus characteristics. The proposed self-supervised models can serve as reference to support other domain-related issues through transfer or multi-task learning paradigms, like the detection and evaluation of the retinal structures or anomaly detections in the context of pathological analysis.
Palabras clave
Component
Formatting
Insert
Style
Styling
Formatting
Insert
Style
Styling
Descripción
© 2021 IEEE. This version of the paper has been accepted for publication. Personal use of this material is permitted. Permission from IEEE must be obtained for all other uses, in any current or future media, including reprinting/republishing this material for advertising or promotional purposes, creating new collective works, for resale or redistribution to servers or lists, or reuse of any copyrighted component of this work in other works. The final published paper is available online at: https://doi.org/10.1109/IJCNN52387.2021.9533567 Proceedings of the International Joint Conference on Neural NetworksVolume 2021-July18 July 2021 2021 International Joint Conference on Neural Networks, IJCNN 2021Virtual, Shenzhen, 18 July 2021 through 22 July 2021, Code 171891
Versión del editor
Derechos
© 2021 IEEE.