Cycle generative adversarial network approaches to produce novel portable chest X-rays images for covid-19 diagnosis
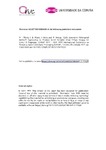
Use este enlace para citar
http://hdl.handle.net/2183/36478Coleccións
- Investigación (FIC) [1678]
Metadatos
Mostrar o rexistro completo do ítemTítulo
Cycle generative adversarial network approaches to produce novel portable chest X-rays images for covid-19 diagnosisData
2021Cita bibliográfica
D. I. Morís, J. de Moura, J. Novo and M. Ortega, "Cycle Generative Adversarial Network Approaches to Produce Novel Portable Chest X-Rays Images for Covid-19 Diagnosis," ICASSP 2021 - 2021 IEEE International Conference on Acoustics, Speech and Signal Processing (ICASSP), Toronto, ON, Canada, 2021, pp. 1060-1064, doi: 10.1109/ICASSP39728.2021.9414031
Resumo
[Abstract]: Coronavirus Disease 2019 (COVID-19), declared a global pandemic by the World Health Organization, mainly affects the pulmonary tissues, playing chest X-ray images an important role for its screening and early detection. In this context, portable X-ray devices are widely used, representing an alternative to fixed devices in order to reduce risks of cross-contamination. However, they provide lower quality and detailed images in terms of spatial resolution and contrast. In this work, given the low availability of images of this recent disease, we present new approaches to artificially increase the dimensionality of portable chest X-ray datasets for COVID-19 diagnosis. Hence, we combined 3 complementary CycleGAN architectures to perform a simultaneous oversampling using an unsupervised strategy and without the necessity of paired data. Despite the poor quality of the portable X-ray images, we provide an overall accuracy of 92.50% in a COVID-19 screening context, proving their suitability for COVID-19 diagnostic tasks.
Palabras chave
COVID-19
CycleGAN
Deep learning
Oversampling
Portable chest X-ray images
CycleGAN
Deep learning
Oversampling
Portable chest X-ray images
Descrición
© 2021 IEEE. This version of the paper has been accepted for publication. Personal use of this material is permitted. Permission from IEEE must be obtained for all other uses, in any current or future media, including reprinting/republishing this material for advertising or promotional purposes, creating new collective works, for resale or redistribution to servers or lists, or reuse of any copyrighted component of this work in other works. The final published paper is available online at: https://doi.org/10.1109/ICASSP39728.2021.9414031 Presentado en: ICASSP 2021 - 2021 IEEE International Conference on Acoustics, Speech and Signal Processing (ICASSP), Toronto, ON, Canada, 2021
Versión do editor
Dereitos
© 2021 IEEE.