CUDA acceleration of MI-based feature selection methods
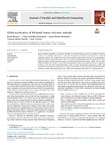
Use este enlace para citar
http://hdl.handle.net/2183/36386
Excepto si se señala otra cosa, la licencia del ítem se describe como Atribución-NoComercial-SinDerivadas 4.0 Internacional
Colecciones
- Investigación (FIC) [1685]
Metadatos
Mostrar el registro completo del ítemTítulo
CUDA acceleration of MI-based feature selection methodsAutor(es)
Fecha
2024-08Cita bibliográfica
Beceiro, B., González-Domínguez, J., Morán-Fernández, L., Bolón-Canedo, V., & Touriño, J. (2024). CUDA acceleration of MI-based feature selection methods. Journal of Parallel and Distributed Computing, 104901. https://doi.org/10.1016/j.jpdc.2024.104901
Resumen
[Abstract]: Feature selection algorithms are necessary nowadays for machine learning as they are capable of removing irrelevant and redundant information to reduce the dimensionality of the data and improve the quality of subsequent analyses. The problem with current feature selection approaches is that they are computationally expensive when processing large datasets. This work presents parallel implementations for Nvidia GPUs of three highly-used feature selection methods based on the Mutual Information (MI) metric: mRMR, JMI and DISR. Publicly available code includes not only CUDA implementations of the general methods, but also an adaptation of them to work with low-precision fixed point in order to further increase their performance on GPUs. The experimental evaluation was carried out on two modern Nvidia GPUs (Turing T4 and Ampere A100) with highly satisfactory results, achieving speedups of up to 283x when compared to state-of-the-art C implementations.
Palabras clave
Feature selection
Mutual information
Low precision
Fixed point
CUDA
Mutual information
Low precision
Fixed point
CUDA
Versión del editor
Derechos
Atribución-NoComercial-SinDerivadas 4.0 Internacional
ISSN
0743-7315
1096-0848
1096-0848