BigDEC: A multi-algorithm Big Data tool based on the k-mer spectrum method for scalable short-read error correction
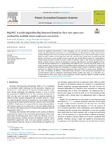
Use este enlace para citar
http://hdl.handle.net/2183/36250
A non ser que se indique outra cousa, a licenza do ítem descríbese como Atribución-NoComercial-SinDerivadas 3.0 España
Coleccións
- Investigación (FIC) [1678]
Metadatos
Mostrar o rexistro completo do ítemTítulo
BigDEC: A multi-algorithm Big Data tool based on the k-mer spectrum method for scalable short-read error correctionData
2024-05Cita bibliográfica
R. R. Expósito, J. González-Domínguez, "BigDEC: A multi-algorithm Big Data tool based on the k-mer spectrum method for scalable short-read error correction", Future Generation Computer Systems, Vol. 154, May 2024, pp. 314 - 329, doi: 10.1016/j.future.2024.01.011
Resumo
[Abstract]: Despite the significant improvements in both throughput and cost provided by modern Next-Generation Sequencing (NGS) platforms, sequencing errors in NGS datasets can still degrade the quality of downstream analysis. Although state-of-the-art correction tools can provide high accuracy to improve such analysis, they are limited to apply a single correction algorithm while also requiring long runtimes when processing large NGS datasets. Furthermore, current parallel correctors generally only provide efficient support for shared-memory systems lacking the ability to scale out across a cluster of multicore nodes, or they require the availability of specific hardware devices or features. In this paper we present a Big Data Error Correction (BigDEC) tool that overcomes all those limitations by: (1) implementing three different error correction algorithms based on the widely extended k-mer spectrum method; (2) providing scalable performance for large datasets by efficiently exploiting the capabilities of Big Data technologies on multicore clusters based on commodity hardware; (3) supporting two different Big Data processing frameworks (Spark and Flink) to provide greater flexibility to end users; (4) including an efficient, stream-based merge operation to ease downstream processing of the corrected datasets; and (5) significantly outperforming existing parallel tools, being up to 79% faster on a 16-node multicore cluster when using the same underlying correction algorithm. BigDEC is publicly available to download at https://github.com/UDC-GAC/BigDEC.
Palabras chave
Apache flink
Apache spark
Big data processing
Error correction
Next generation sequencing (NGS)
Apache spark
Big data processing
Error correction
Next generation sequencing (NGS)
Descrición
Financiado para publicación en acceso aberto: Universidade da Coruña/CISUG
Versión do editor
Dereitos
Atribución-NoComercial-SinDerivadas 3.0 España