Evolutionary multi-target neural network architectures for flow void analysis in optical coherence tomography angiography
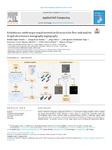
Ver/Abrir
Use este enlace para citar
http://hdl.handle.net/2183/36204
Excepto si se señala otra cosa, la licencia del ítem se describe como Atribución-NoComercial-SinDerivadas 4.0 Internacional
Colecciones
- Investigación (FIC) [1678]
Metadatos
Mostrar el registro completo del ítemTítulo
Evolutionary multi-target neural network architectures for flow void analysis in optical coherence tomography angiographyAutor(es)
Fecha
2024-03Cita bibliográfica
López-Varela, E., de Moura, J., Novo, J., Fernández-Vigo, J. I., Moreno-Morillo, F. J., García-Feijóo, J., & Ortega, M. (2024). Evolutionary multi-target neural network architectures for flow void analysis in optical coherence tomography angiography. Applied Soft Computing, 153, 111304.
Resumen
[Abstract]: Optical coherence tomography angiography (OCTA) is a non-invasive imaging modality used to evaluate the retinal microvasculature. Recent advances in OCTA allows to visualize the blood flow within the choriocapillaris region, where a granular image is obtained showing a pattern of small dark regions, called flow voids (FVs). Given its relevance, numerous clinical studies have linked the changes in FVs distribution to multiple diseases. The granular structure of these images makes accurate labeling and segmentation difficult, which can be overcome by using a multi-target perspective. However, manually designing a neural architecture that can accurately predict all targets in a balanced way is a major challenge. In this work, we propose a novel methodology based on evolutionary multi-target optimized networks that, through a set of evolutionary operators, traverses a search space of architectures in a deep but efficient way. This methodology allows us to discover efficient and accurate multi-target architectures tailored to our problem, but which are also adaptable to other tasks due to their robustness. To validate and analyze our methodology and the discovered network model, we performed extensive experimentation with cases from a real clinical study, achieving better results than the state of the art and manually designed architectures.
Palabras clave
Central serous chorioretinopathy
Evolutionary neural networks
Flow voids
Multi-target
OCTA imaging
Evolutionary neural networks
Flow voids
Multi-target
OCTA imaging
Versión del editor
Derechos
Atribución-NoComercial-SinDerivadas 4.0 Internacional
ISSN
1568-4946
1872-9681
1872-9681