Multi-Adaptive Optimization for multi-task learning with deep neural networks
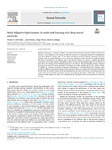
Use este enlace para citar
http://hdl.handle.net/2183/36191
A non ser que se indique outra cousa, a licenza do ítem descríbese como Atribución-NoComercial-SinDerivadas 3.0 España
Coleccións
- Investigación (FIC) [1679]
Metadatos
Mostrar o rexistro completo do ítemTítulo
Multi-Adaptive Optimization for multi-task learning with deep neural networksData
2024-02Cita bibliográfica
Á. S. Hervella, J. Rouco, J. Novo, and M. Ortega, "Multi-Adaptive Optimization for multi-task learning with deep neural networks ", Neural Networks, Vol. 170, Pp. 254-265, Feb. 2024, doi: 10.1016/j.neunet.2023.11.038
Resumo
[Abstract]: Multi-task learning is a promising paradigm to leverage task interrelations during the training of deep neural networks. A key challenge in the training of multi-task networks is to adequately balance the complementary supervisory signals of multiple tasks. In that regard, although several task-balancing approaches have been proposed, they are usually limited by the use of per-task weighting schemes and do not completely address the uneven contribution of the different tasks to the network training. In contrast to classical approaches, we propose a novel Multi-Adaptive Optimization (MAO) strategy that dynamically adjusts the contribution of each task to the training of each individual parameter in the network. This automatically produces a balanced learning across tasks and across parameters, throughout the whole training and for any number of tasks. To validate our proposal, we perform comparative experiments on real-world datasets for computer vision, considering different experimental settings. These experiments allow us to analyze the performance obtained in several multi-task scenarios along with the learning balance across tasks, network layers and training steps. The results demonstrate that MAO outperforms previous task-balancing alternatives. Additionally, the performed analyses provide insights that allow us to comprehend the advantages of this novel approach for multi-task learning.
Palabras chave
Computer vision
Deep learning
Gradient descent
Multi-task learning
Neural networks
Optimization
Deep learning
Gradient descent
Multi-task learning
Neural networks
Optimization
Descrición
Financiado para publicación en acceso aberto: Universidade da Coruña/CISUG
Versión do editor
Dereitos
Atribución-NoComercial-SinDerivadas 3.0 España