Multi-Adaptive Optimization for multi-task learning with deep neural networks
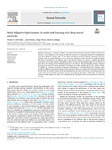
Use this link to cite
http://hdl.handle.net/2183/36191
Except where otherwise noted, this item's license is described as Atribución-NoComercial-SinDerivadas 3.0 España
Collections
- GI-VARPA - Artigos [76]
Metadata
Show full item recordTitle
Multi-Adaptive Optimization for multi-task learning with deep neural networksDate
2024-02Citation
Á. S. Hervella, J. Rouco, J. Novo, and M. Ortega, "Multi-Adaptive Optimization for multi-task learning with deep neural networks ", Neural Networks, Vol. 170, Pp. 254-265, Feb. 2024, doi: 10.1016/j.neunet.2023.11.038
Abstract
[Abstract]: Multi-task learning is a promising paradigm to leverage task interrelations during the training of deep neural networks. A key challenge in the training of multi-task networks is to adequately balance the complementary supervisory signals of multiple tasks. In that regard, although several task-balancing approaches have been proposed, they are usually limited by the use of per-task weighting schemes and do not completely address the uneven contribution of the different tasks to the network training. In contrast to classical approaches, we propose a novel Multi-Adaptive Optimization (MAO) strategy that dynamically adjusts the contribution of each task to the training of each individual parameter in the network. This automatically produces a balanced learning across tasks and across parameters, throughout the whole training and for any number of tasks. To validate our proposal, we perform comparative experiments on real-world datasets for computer vision, considering different experimental settings. These experiments allow us to analyze the performance obtained in several multi-task scenarios along with the learning balance across tasks, network layers and training steps. The results demonstrate that MAO outperforms previous task-balancing alternatives. Additionally, the performed analyses provide insights that allow us to comprehend the advantages of this novel approach for multi-task learning.
Keywords
Computer vision
Deep learning
Gradient descent
Multi-task learning
Neural networks
Optimization
Deep learning
Gradient descent
Multi-task learning
Neural networks
Optimization
Description
Financiado para publicación en acceso aberto: Universidade da Coruña/CISUG
Editor version
Rights
Atribución-NoComercial-SinDerivadas 3.0 España