Twitter: A Good Place to Detect Health Conditions
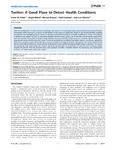
Use este enlace para citar
http://hdl.handle.net/2183/35044Coleccións
- Investigación (FIC) [1678]
Metadatos
Mostrar o rexistro completo do ítemTítulo
Twitter: A Good Place to Detect Health ConditionsAutor(es)
Data
2014-01Cita bibliográfica
Prieto VM, Matos S, Álvarez M, Cacheda F, Oliveira JL (2014) Twitter: A Good Place to Detect Health Conditions. PLoS ONE 9(1): e86191. https://doi.org/10.1371/journal.pone.0086191
Resumo
[Absctract]: With the proliferation of social networks and blogs, the Internet is increasingly being used to disseminate personal health information rather than just as a source of information. In this paper we exploit the wealth of user-generated data, available through the micro-blogging service Twitter, to estimate and track the incidence of health conditions in society. The method is based on two stages: we start by extracting possibly relevant tweets using a set of specially crafted regular expressions, and then classify these initial messages using machine learning methods. Furthermore, we selected relevant features to improve the results and the execution times. To test the method, we considered four health states or conditions, namely flu, depression, pregnancy and eating disorders, and two locations, Portugal and Spain.
We present the results obtained and demonstrate that the detection results and the performance of the method are improved after feature selection. The results are promising, with areas under the receiver operating characteristic curve between 0.7 and 0.9, and f-measure values around 0.8 and 0.9. This fact indicates that such approach provides a feasible solution for measuring and tracking the evolution of health states within the society.
Palabras chave
Twitter
Influenza
Depression
Eating disorders
Epidemiology
Machine learning
Pregnancy
Data mining
Influenza
Depression
Eating disorders
Epidemiology
Machine learning
Pregnancy
Data mining
Versión do editor
Dereitos
Atribución 3.0 España
ISSN
1932-6203