Multithreaded and Spark parallelization of feature selection filters
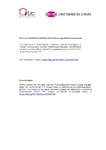
Use este enlace para citar
http://hdl.handle.net/2183/34589
Excepto si se señala otra cosa, la licencia del ítem se describe como Atribución-NoComercial-SinDerivadas 3.0 España
Colecciones
- Investigación (FIC) [1679]
Metadatos
Mostrar el registro completo del ítemTítulo
Multithreaded and Spark parallelization of feature selection filtersAutor(es)
Fecha
2016Cita bibliográfica
C. Eiras-Franco, V. Bolón-Canedo, S. Ramos, J. González-Domínguez, A. Alonso-Betanzos, and J. Touriño, "Multithreaded and Spark parallelization of feature selection filters", Journal of Computational Science, Vol. 17, Part 3, Nov. 2016, Pp. 609-619, https://doi.org/10.1016/j.jocs.2016.07.002
Es versión de
https://doi.org/10.1016/j.jocs.2016.07.002
Resumen
[Abstract]: Vast amounts of data are generated every day, constituting a volume that is challenging to analyze. Techniques such as feature selection are advisable when tackling large datasets. Among the tools that provide this functionality, Weka is one of the most popular ones, although the implementations it provides struggle when processing large datasets, requiring excessive times to be practical. Parallel processing can help alleviate this problem, effectively allowing users to work with Big Data. The computational power of multicore machines can be harnessed by using multithreading and distributed programming, effectively helping to tackle larger problems. Both these techniques can dramatically speed up the feature selection process allowing users to work with larger datasets. The reimplementation of four popular feature selection algorithms included in Weka is the focus of this work. Multithreaded implementations previously not included in Weka as well as parallel Spark implementations were developed for each algorithm. Experimental results obtained from tests on real-world datasets show that the new versions offer significant reductions in processing times.
Palabras clave
Multithreading
Spark
Feature selection
Machine learning
Spark
Feature selection
Machine learning
Descripción
©2016 Elsevier B.V. All rights reserved. This manuscript version is made available under the CC-BY-NC-ND 4.0 license https://creativecommons.org/licenses/bync-nd/4.0/. This version of the article has been accepted for publication in Journal of Computational Science. The Version of Record is available online at https://doi.org/10.1016/j.jocs.2016.07.002 Versión final aceptada de: C. Eiras-Franco, V. Bolón-Canedo, S. Ramos, J. González-Domínguez, A. Alonso-Betanzos, and J. Touriño, "Multithreaded and Spark parallelization of feature selection filters", Journal of Computational Science, Vol. 17, Part 3, Nov. 2016, Pp. 609-619
Versión del editor
Derechos
Atribución-NoComercial-SinDerivadas 3.0 España