Q-Learning based system for Path Planning with Unmanned Aerial Vehicles swarms in obstacle environments
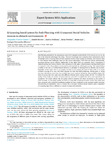
Use this link to cite
http://hdl.handle.net/2183/34437
Except where otherwise noted, this item's license is described as Attribution-NonCommercial-NoDerivs 4.0 International (CC BY-NC-ND)
Collections
- Investigación (FIC) [1681]
Metadata
Show full item recordTitle
Q-Learning based system for Path Planning with Unmanned Aerial Vehicles swarms in obstacle environmentsAuthor(s)
Date
2023Citation
Puente-Castro, A., Rivero, D., Pedrosa, E., Pereira, A., Lau, N., & Fernandez-Blanco, E. (2023). Q-Learning based system for Path Planning with Unmanned Aerial Vehicles swarms in obstacle environments. Expert Systems With Applications, 235, 121240.https://doi.org/10.1016/j.eswa.2023.121240
Abstract
[Abstract]: Path Planning methods for the autonomous control of Unmanned Aerial Vehicle (UAV) swarms are on the rise due to the numerous advantages they bring. There are increasingly more scenarios where autonomous control of multiple UAVs is required. Most of these scenarios involve a large number of obstacles, such as power lines or trees. Despite these challenges, there are also several advantages; if all UAVs can operate autonomously, personnel expenses can be reduced. Additionally, if their flight paths are optimized, energy consumption is reduced, leaving more battery time for other operations. In this paper, a Reinforcement Learning-based system is proposed to solve this problem in environments with obstacles by utilizing Q-Learning. This method allows a model, in this case, an Artificial Neural Network, to self-adjust by learning from its mistakes and successes. Regardless of the map’s size or the number of UAVs in the swarm, the goal of these paths is to ensure complete coverage of an area with fixed obstacles for tasks like field prospecting. Setting goals or having any prior information apart from the provided map is not required. During the experimentation phase, five maps of varying sizes were used, each with different obstacles and a varying number of UAVs. To evaluate the quality of the results, the number of actions taken by each UAV to complete the task in each experiment was considered. The results indicate that the system achieves solutions with fewer movements as the number of UAVs increases. An increasing number of UAVs on a map lead to solutions in fewer moves. The results have been compared, and a statistical significance analysis has been conducted on the proposed model’s outcomes, demonstrating its capabilities. Thus, it is shown that a two-layer Artificial Neural Network used to implement a Q-Learning algorithm is sufficient to operate on maps with obstacles.
Keywords
UAV
Artificial neural network
Reinforcement learning
Path planning
Obstacle
Swarm
Artificial neural network
Reinforcement learning
Path planning
Obstacle
Swarm
Editor version
Rights
Attribution-NonCommercial-NoDerivs 4.0 International (CC BY-NC-ND)