Predicting Inflow Flow in Hydraulic Dams Using Artificial Neural Networks
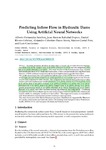
Use this link to cite
http://hdl.handle.net/2183/34239
Except where otherwise noted, this item's license is described as Attribution 4.0 International (CC BY 4.0)
Metadata
Show full item recordTitle
Predicting Inflow Flow in Hydraulic Dams Using Artificial Neural NetworksAuthor(s)
Date
2023Abstract
[Abstract] Accurate prediction of inflow in dams plays a crucial role in water resource management Kim et al. (2019); Vargas-Garay et al. (2018); Zhong et al. (2018) and risk mitigation Costabile et al. (2020); Rabuñal et al. (2007). This study focuses on the Portodemouros dam (located between the provinces of A Coruña and Pontevedra), where a model based on a Long Short-Term Memory (LSTM) artificial neural network has been implemented to predict dam inflow. The results demonstrate the well-established effectiveness of the LSTM network in flow prediction Dongkyuna and Seokkoob (2021); Jo and Jung (2023); Li et al. (2020) applied to the Portodemouros dam compared to other models. This comparison has already been performed in other studies with both mathematical models Amirreza et al. (2022); Ansori and Anwar (2022); A.R1 et al. (2018); Beck et al. (2017); Ciabatta et al. (2016); Costabile et al. (2020); Fan et al. (2013); Hermanovsky et al. (2017); Kim et al. (2019); Vargas-Garay et al. (2018); Zhong et al. (2018), genetic programming Aytek et al. (2008); Havl´ıˇcek et al. (2013); Heˇrmanovsk´y et al. (2017); Rabuñal et al. (2007) and other machine learning algorithms Jo and Jung (2023). Combining precipitation data from multiple regions and meteorological forecasts significantly enhances the model’s ability to anticipate variations in dam inflow. This improved accuracy is essential for early flood detection and informed decision-making in dam operation. This study forms part of the Marine Science programme (ThinkInAzul) supported by Ministerio de Ciencia e Innovación and Xunta de Galicia with funding from European Union NextGenerationEU (PRTR-C17.I1) and European Maritime and Fisheries Fund
Keywords
Presa de Portodemouros
Predicción de caudales
Modelos matemáticos
Predicción de caudales
Modelos matemáticos
Description
Cursos e Congresos, C-155
Editor version
Rights
Attribution 4.0 International (CC BY 4.0)