A Scalable Approach to Independent Vector Analysis by Shared Subspace Separation for Multi-Subject fMRI Analysis
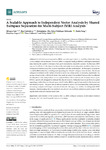
View/ Open
Use this link to cite
http://hdl.handle.net/2183/34231Collections
- Investigación (FIC) [1685]
Metadata
Show full item recordTitle
A Scalable Approach to Independent Vector Analysis by Shared Subspace Separation for Multi-Subject fMRI AnalysisAuthor(s)
Date
2023-06Citation
M. Sun et al., “A Scalable Approach to Independent Vector Analysis by Shared Subspace Separation for Multi-Subject fMRI Analysis,” Sensors, vol. 23, no. 11, p. 5333, Jun. 2023, doi: 10.3390/s23115333
Abstract
[Abstract]: Joint blind source separation (JBSS) has wide applications in modeling latent structures across multiple related datasets. However, JBSS is computationally prohibitive with high-dimensional data, limiting the number of datasets that can be included in a tractable analysis. Furthermore, JBSS may not be effective if the data’s true latent dimensionality is not adequately modeled, where severe overparameterization may lead to poor separation and time performance. In this paper, we propose a scalable JBSS method by modeling and separating the “shared” subspace from the data. The shared subspace is defined as the subset of latent sources that exists across all datasets, represented by groups of sources that collectively form a low-rank structure. Our method first provides the efficient initialization of the independent vector analysis (IVA) with a multivariate Gaussian source prior (IVA-G) specifically designed to estimate the shared sources. Estimated sources are then evaluated regarding whether they are shared, upon which further JBSS is applied separately to the shared and non-shared sources. This provides an effective means to reduce the dimensionality of the problem, improving analyses with larger numbers of datasets. We apply our method to resting-state fMRI datasets, demonstrating that our method can achieve an excellent estimation performance with significantly reduced computational costs.
Keywords
Functional magnetic resonance imaging
Independent vector analysis
JBSS
MCCA
Multi-subject medical imaging data
Subspace analysis
Independent vector analysis
JBSS
MCCA
Multi-subject medical imaging data
Subspace analysis
Editor version
Rights
Atribución 4.0 Internacional