Artificial neural network prediction of the initial stiffness of semi-rigid beam-to-column connections
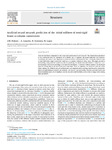
Use this link to cite
http://hdl.handle.net/2183/34171
Except where otherwise noted, this item's license is described as Attribution-NonCommercial-NoDerivs 4.0 International
Collections
Metadata
Show full item recordTitle
Artificial neural network prediction of the initial stiffness of semi-rigid beam-to-column connectionsDate
2023-10Citation
Reinosa, J. M., A. Loureiro, R. Gutierrez, and M. Lopez. 2023. “Artificial Neural Network Prediction of the Initial Stiffness of Semi-Rigid Beam-to-Column Connections,” Structures 56, 56: 104904. https://doi.org/10.1016/j.istruc.2023.104904.
Abstract
[Abstract]: Joints are significant components in the design and construction of steel structures. The characteristic parameters of the connections must be reproduced in a reliable way to represent the actual behaviour of a structure. Accordingly, the study of semi-rigid joints is essential to better understand this issue. Among the different types of semi-rigid joints, angle connections stand out as a suitable solution in many cases. This paper presents a methodology using artificial neural networks for predicting the initial rotational stiffness of major axis symmetrical angle connections according to the Eurocode description. A consistent stiffness database was developed from the existing data in the Steel Connection Data Bank. Then, the database was cleansed to provide with a robust training set. Different network architectures were analysed until a topology that showed a good performance and generalisation features was obtained. The network was successfully checked with some saved tests from the database and with off-database tests; the network could be reliably used within the range of the training input parameters.
Keywords
Angle connections
Semi-rigid joints
Initial stiffness
Artificial neural networks
Semi-rigid joints
Initial stiffness
Artificial neural networks
Editor version
Rights
Attribution-NonCommercial-NoDerivs 4.0 International
ISSN
2352-0124