Towards a FAIR Dataset for Spanish Non-Functional Requirements
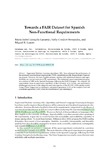
Use este enlace para citar
http://hdl.handle.net/2183/34160
Excepto si se señala otra cosa, la licencia del ítem se describe como Attribution 4.0 International (CC BY 4.0)
Metadatos
Mostrar el registro completo del ítemTítulo
Towards a FAIR Dataset for Spanish Non-Functional RequirementsFecha
2023Resumen
[Abstract] Supervised Machine Learning algorithms (ML) have enhanced the performance of
the automatic non-functional requirements (NFR) classification in the Requirements Engineering
domain. However, the lack of public datasets, dealing with imbalanced datasets and reproducibility
are current concerns in ML experiments. We conducted a quasi-experiment to generate
a dataset of NFR in the Spanish Language, following the FAIR Principles. We collected 109
requirements from an open access repository of the University of A Coru˜ na, and performed a
labeling process based in the categories and subcategories of the ISO/IEC 25010 quality model.
Using a Fleiss’ Kappa test we obtained a substantial agreement (0.78) at the category level and
a moderate agreement (0.48) when the classification is per subcategory supervised Machine Learning algorithms (ML) have enhanced the performance of the automatic non-functional requirements (NFR) classification in the Requirements Engineering domain. However, the lack of public datasets, dealing with imbalanced datasets and reproducibility are current concerns in ML experiments. We conducted a quasi-experiment to generate
a dataset of NFR in the Spanish Language, following the FAIR Principles. We collected 109
requirements from an open access repository of the University of A Coruña, and performed a
labeling process based in the categories and subcategories of the ISO/IEC 25010 quality model.
Using a Fleiss’ Kappa test we obtained a substantial agreement (0.78) at the category level and a moderate agreement (0.48) when the classification is per subcategory
Palabras clave
Aprendizaje automático
Principios FAIR
Kappa de Fleiss
Principios FAIR
Kappa de Fleiss
Descripción
Cursos e Congresos, C-155
Versión del editor
Derechos
Attribution 4.0 International (CC BY 4.0)