LyS A Coruña at GUA-SPA@IberLEF2023. Multi-Task Learning with Large Language Model Encoders for Guarani-Spanish Code Switching Analysis
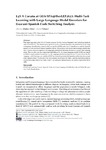
Use this link to cite
http://hdl.handle.net/2183/33478Collections
- GI-LYS - Congresos, conferencias, etc. [36]
- OpenAIRE [266]
Metadata
Show full item recordTitle
LyS A Coruña at GUA-SPA@IberLEF2023. Multi-Task Learning with Large Language Model Encoders for Guarani-Spanish Code Switching AnalysisDate
2023Citation
A. Muñoz Ortiz, D. Vilares. LyS A Coruña at GUA-SPA@IberLEF2023: Multi-Task Learning with Large Language Model Encoders for Guarani-Spanish Code Switching Analysis, in: Proceedings of IberLEF 2023, Jaén, Spain
Abstract
[Abstract] This paper introduces the LyS A Coruña proposal for the Guarani-Spanish Code Switching Analysis
task at IberLEF2023. The shared task proposes to analyze Guarani-Spanish code-switched texts, focusing
on language identification, named entity recognition (NER), and a novel classification task for Spanish
spans in a code-switched Guarani-Spanish context. We propose three multi-task learning systems that
have common encoders based on two language models and different decoders in a multi-task learning
setup. The encoders use the contextual embeddings by: (i) a large language model (LLM) pretrained
on bidirectional machine translation on 200 languages (including Spanish and Guarani) from the No
Language Left Behind project, and (ii) a BERT-based model pretrained in Spanish and finetuned in around
800k Guarani tokens. The decoders are: (i) a softmax output layer for Task 1, and (ii) conditional random
fields (CRF) output layers for Tasks 2 and 3. According to official results, we ranked third in the three
tasks.
Keywords
Multi-Task Learning
Guaraní
Spanish
Code-switching
Language identification
Named Entity Recognition
Code Classification,
Guaraní
Spanish
Code-switching
Language identification
Named Entity Recognition
Code Classification,
Rights
Atribución 4.0 Internacional