A novel method for anomaly detection using beta Hebbian learning and principal component analysis
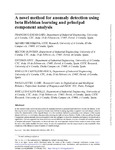
Use this link to cite
http://hdl.handle.net/2183/33050
Except where otherwise noted, this item's license is described as Attribution 4.0 International (CC BY 4.0) https://creativecommons.org/licenses/by/4.0/
Collections
- Investigación (EPEF) [590]
Metadata
Show full item recordTitle
A novel method for anomaly detection using beta Hebbian learning and principal component analysisAuthor(s)
Date
2023-04Citation
Zayas-Gato F, Michelena Á, Quintián H et al. A novel method for anomaly detection using beta Hebbian learning and principal component analysis. Logic Journal of the IGPL 2023;31:390–9.
Abstract
[Abstract] In this research work a novel two-step system for anomaly detection is presented and tested over several real datasets. In the first step the novel Exploratory Projection Pursuit, Beta Hebbian Learning algorithm, is applied over each dataset, either to reduce the dimensionality of the original dataset or to face nonlinear datasets by generating a new subspace of the original dataset with lower, or even higher, dimensionality selecting the right activation function. Finally, in the second step Principal Component Analysis anomaly detection is applied to the new subspace to detect the anomalies and improve its classification capabilities. This new approach has been tested over several different real datasets, in terms of number of variables, number of samples and number of anomalies. In almost all cases, the novel approach obtained better results in terms of area under the curve with similar standard deviation values. In case of computational cost, this improvement is only remarkable when complexity of the dataset in terms of number of variables is high.
Keywords
One-class
Dimensional reduction
BHL
PCA
Dimensional reduction
BHL
PCA
Editor version
Rights
Attribution 4.0 International (CC BY 4.0) https://creativecommons.org/licenses/by/4.0/
ISSN
1368-9894