Local Correlation Integral Approach for Anomaly Detection Using Functional Data
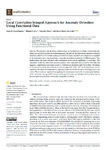
Use este enlace para citar
http://hdl.handle.net/2183/32830Colecciones
- GI-MODES - Artigos [122]
Metadatos
Mostrar el registro completo del ítemTítulo
Local Correlation Integral Approach for Anomaly Detection Using Functional DataFecha
2023-02-06Cita bibliográfica
Sosa Donoso, J.R.; Flores, M.; Naya, S.; Tarrío-Saavedra, J. Local Correlation Integral Approach for Anomaly Detection Using Functional Data. Mathematics 2023, 11, 815. https://doi.org/10.3390/math11040815
Resumen
[Abstract]: The present work develops a methodology for the detection of outliers in functional data, taking into account both their shape and magnitude. Specifically, the multivariate method of anomaly detection called Local Correlation Integral (LOCI) has been extended and adapted to be applied to the particular case of functional data, using the calculation of distances in Hilbert spaces. This methodology has been validated with a simulation study and its application to real data. The simulation study has taken into account scenarios with functional data or curves with different degrees of dependence, as is usual in cases of continuously monitored data versus time. The results of the simulation study show that the functional approach of the LOCI method performs well in scenarios with inter-curve dependence, especially when the outliers are due to the magnitude of the curves. These results are supported by applying the present procedure to the meteorological database of the Alternative Energy and Environment Group in Ecuador, specifically to the humidity curves, presenting better performance than other competitive methods.
Palabras clave
anomaly detection
FDA
Hilbert space
LOCI
outlier detection
FDA
Hilbert space
LOCI
outlier detection
Versión del editor
Derechos
Atribución 3.0 España
ISSN
2227-7390