Boundary-safe PINNs extension: Application to non-linear parabolic PDEs in counterparty credit risk
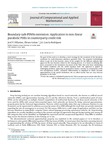
View/ Open
Use this link to cite
http://hdl.handle.net/2183/32780
Except where otherwise noted, this item's license is described as Atribución-NoComercial-SinDerivadas 4.0 International (CC BY-NC-ND 4.0)
Collections
- Investigación (FIC) [1685]
Metadata
Show full item recordTitle
Boundary-safe PINNs extension: Application to non-linear parabolic PDEs in counterparty credit riskDate
2023Citation
J. P. Villarino, Á. Leitao, & J.A. García Rodríguez, "Boundary-safe PINNs extension: Application to non-linear parabolic PDEs in counterparty credit risk", Journal of Computational and Applied Mathematics, 425, 2023 [Online] doi:10.1016/j.cam.2022.115041, Available: https://doi.org/10.1016/j.cam.2022.115041
Abstract
[Abstract]: The goal of this work is to develop a novel strategy for the treatment of the boundary conditions for multi-dimension nonlinear parabolic PDEs. The proposed methodology allows to get rid of the heuristic choice of the weights for the different addends that appear in the loss function related to the training process. It is based on defining the losses associated to the boundaries by means of the PDEs that arise from substituting the related conditions into the model equation itself. The approach is applied to challenging problems appearing in quantitative finance, namely, in counterparty credit risk management. Further, automatic differentiation is employed to obtain accurate approximation of the partial derivatives, the so called Greeks, that are very relevant quantities in the field.
Keywords
Boundary conditions
Couterparty credit risk
Deep learning
Nonlinear
PDEs
PINNs
Couterparty credit risk
Deep learning
Nonlinear
PDEs
PINNs
Editor version
Rights
Atribución-NoComercial-SinDerivadas 4.0 International (CC BY-NC-ND 4.0)