Transition-based semantic role labeling with pointer networks
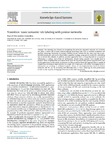
Use this link to cite
http://hdl.handle.net/2183/32764
Except where otherwise noted, this item's license is described as Atribución-NoComercial-SinDerivadas 4.0 Internacional
Collections
- Investigación (FFIL) [877]
Metadata
Show full item recordTitle
Transition-based semantic role labeling with pointer networksAuthor(s)
Date
2023-01-25Citation
Fernández-González, Daniel (2023) : Transition-based semantic role labeling with pointer networks. Knowledge-Based Systems, vol. 260, 110127
Abstract
[Abstract] Semantic role labeling (SRL) focuses on recognizing the predicate–argument structure of a sentence and plays a critical role in many natural language processing tasks such as machine translation and question answering. Practically all available methods do not perform full SRL, since they rely on pre-identified predicates, and most of them follow a pipeline strategy, using specific models for undertaking one or several SRL subtasks. In addition, previous approaches have a strong dependence on syntactic information to achieve state-of-the-art performance, despite being syntactic trees equally hard to produce. These simplifications and requirements make the majority of SRL systems impractical for real-world applications. In this article, we propose the first transition-based SRL approach that is capable of completely processing an input sentence in a single left-to-right pass, with neither leveraging syntactic information nor resorting to additional modules. Thanks to our implementation based on Pointer Networks, full SRL can be accurately and efficiently done in
, achieving the best performance to date on the majority of languages from the CoNLL-2009 shared task.
Keywords
Natural language processing
Computational linguistics
Semantic role labeling
Neural networks
Deep learning
Computational linguistics
Semantic role labeling
Neural networks
Deep learning
Editor version
Rights
Atribución-NoComercial-SinDerivadas 4.0 Internacional
ISSN
0950-7051