Comparison of machine learning techniques for reservoir outflow forecasting
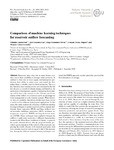
Use este enlace para citar
http://hdl.handle.net/2183/32747Coleccións
- Investigación (ETSECCP) [826]
Metadatos
Mostrar o rexistro completo do ítemTítulo
Comparison of machine learning techniques for reservoir outflow forecastingAutor(es)
Data
2022Cita bibliográfica
García-Feal, O., González-Cao, J., Fernández-Nóvoa, D., Astray Dopazo, G., Gómez-Gesteira, M.: Comparison of machine learning techniques for reservoir outflow forecasting, Nat. Hazards Earth Syst. Sci., 22, 3859–3874, https://doi.org/10.5194/nhess-22-3859-2022, 2022.
Resumo
[Abstract:] Reservoirs play a key role in many human societies due to their capability to manage water resources. In addition to their role in water supply and hydropower production, their ability to retain water and control the flow makes them a valuable asset for flood mitigation. This is a key function, since extreme events have increased in the last few decades as a result of climate change, and therefore, the application of mechanisms capable of mitigating flood damage will be key in the coming decades. Having a good estimation of the outflow of a reservoir can be an advantage for water management or early warning systems. When historical data are available, data-driven models have been proven a useful tool for different hydrological applications. In this sense, this study analyzes the efficiency of different machine learning techniques to predict reservoir outflow, namely multivariate linear regression (MLR) and three artificial neural networks: multilayer perceptron (MLP), nonlinear autoregressive exogenous (NARX) and long short-term memory (LSTM). These techniques were applied to forecast the outflow of eight water reservoirs of different characteristics located in the Miño River (northwest of Spain). In general, the results obtained showed that the proposed models provided a good estimation of the outflow of the reservoirs, improving the results obtained with classical approaches such as to consider reservoir outflow equal to that of the previous day. Among the different machine learning techniques analyzed, the NARX approach was the option that provided the best estimations on average.
Palabras chave
Reservoir outflow
Water management
Early warning system
Machine learning techniques
Water management
Early warning system
Machine learning techniques
Descrición
Número especial: Advances in machine learning for natural hazards risk assessment
Versión do editor
Dereitos
Atribución 3.0 España