A hybrid one-class approach for detecting anomalies in industrial systems
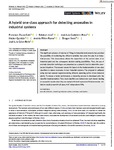
Use este enlace para citar
http://hdl.handle.net/2183/32333
A non ser que se indique outra cousa, a licenza do ítem descríbese como Attribution 4.0 International (CC BY 4.0)
Coleccións
- GI-CTC - Artigos [80]
Metadatos
Mostrar o rexistro completo do ítemTítulo
A hybrid one-class approach for detecting anomalies in industrial systemsAutor(es)
Data
2022-03-08Cita bibliográfica
Zayas-Gato, F., Jove, E., Casteleiro-Roca, J.-L., Quintián, H., Piñ on-Pazos, A., Simi c, D., & Calvo-Rolle, J. L. (2022). A hybrid one-class approach for detecting anomalies in industrial systems. Expert Systems, 39(9), e12990. https://doi.org/10.1111/exsy.12990
Resumo
[Abstract]: The significant advance of Internet of Things in industrial environments has provided the possibility of monitoring the different variables that come into play in an industrial process. This circumstance allows the supervision of the current state of an industrial plant and the consequent decision making possibilities. Then, the use of anomaly detection techniques are presented as a powerful tool to determine unexpected situations. The present research is based on the implementation of one-class classifiers to detect anomalies in two industrial systems. The proposal is validated using two real datasets registered during different operating points of two industrial plants. To ensure a better performance, a clustering process is developed prior the classifier implementation. Then, local classifiers are trained over each cluster, leading to successful results when they are tested with both real and artificial anomalies. Validation results present in all cases, AUC values above 90%.
Palabras chave
Anomaly detection
Clustering
Industrial system
One-class
Optimization
Clustering
Industrial system
One-class
Optimization
Descrición
Financiado para publicación en aberto: Universidade da Coruña/CISUG
Versión do editor
Dereitos
Attribution 4.0 International (CC BY 4.0)
ISSN
1468-0394