Reduced precision discretization based on information theory
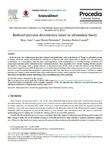
Use this link to cite
http://hdl.handle.net/2183/32305
Except where otherwise noted, this item's license is described as Atribución-NoComercial-SinDerivadas 3.0 España
Collections
- GI-LIDIA - Artigos [65]
Metadata
Show full item recordTitle
Reduced precision discretization based on information theoryDate
2022-01Citation
B. Ares, L. Morán-Fernández, y V. Bolón-Canedo, «Reduced precision discretization based on information theory», Procedia Computer Science, vol. 207, pp. 887-896, ene. 2022, doi: 10.1016/j.procs.2022.09.144.
Abstract
[Abstract] In recent years, new technological areas have emerged and proliferated, such as the Internet of Things or embedded systems in drones, which are usually characterized by making use of devices with strict requirements of weight, size, cost and power consumption. As a consequence, there has been a growing interest in the implementation of machine learning algorithms with reduced precision that can be embedded in these constrained devices. These algorithms cover not only learning, but they can also be applied to other stages such as feature selection or data discretization. In this work we study the behavior of the Minimum Description Length Principle (MDLP) discretizer, proposed by Fayyad and Irani, when reduced precision is used, and how much it affects to a typical machine learning pipeline. Experimental results show that the use of fixed-point format is sufficient to achieve performances similar to those obtained when using double-precision format, which opens the door to the use of reduced-precision discretizers in embedded systems, minimizing energy consumption and carbon emissions.
Keywords
Reduced precision
Discretization
Preprocessing
Mutual information
Machine learning
Discretization
Preprocessing
Mutual information
Machine learning
Editor version
Rights
Atribución-NoComercial-SinDerivadas 3.0 España
ISSN
1877-0509