Compact and indexed representation for LiDAR point clouds
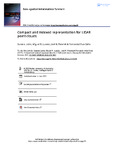
Use this link to cite
http://hdl.handle.net/2183/32253
Except where otherwise noted, this item's license is described as Atribución 4.0 Internacional (CC BY 4.0)
Collections
- Investigación (FIC) [1683]
Metadata
Show full item recordTitle
Compact and indexed representation for LiDAR point cloudsDate
2022Citation
Susana Ladra, Miguel R. Luaces, José R. Paramá & Fernando Silva-Coira (2022): Compact and indexed representation for LiDAR point clouds, Geo-spatial Information Science, DOI: 10.1080/10095020.2022.2121664
Abstract
[Abstract]: LiDAR devices are capable of acquiring clouds of 3D points reflecting any object around them, and adding additional attributes to each point such as color, position, time, etc. LiDAR datasets are usually large, and compressed data formats (e.g. LAZ) have been proposed over the years. These formats are capable of transparently decompressing portions of the data, but they are not focused on solving general queries over the data. In contrast to that traditional approach, a new recent research line focuses on designing data structures that combine compression and indexation, allowing directly querying the compressed data. Compression is used to fit the data structure in main memory all the time, thus getting rid of disk accesses, and indexation is used to query the compressed data as fast as querying the uncompressed data. In this paper, we present the first data structure capable of losslessly compressing point clouds that have attributes and jointly indexing all three dimensions of space and attribute values. Our method is able to run range queries and attribute queries up to 100 times faster than previous methods.
Keywords
3D point clouds
Lossless compression
Indexing
Lossless compression
Indexing
Editor version
Rights
Atribución 4.0 Internacional (CC BY 4.0)